Machine Learning for Science: Mathematics at the Interface of Data-driven and Mechanistic Modelling
Neil Lawrence
University of Cambridge, UKJessica Montgomery
University of Cambridge, UKBernhard Schölkopf
Max Planck Institute for Intelligent Systems, Tübingen, Germany
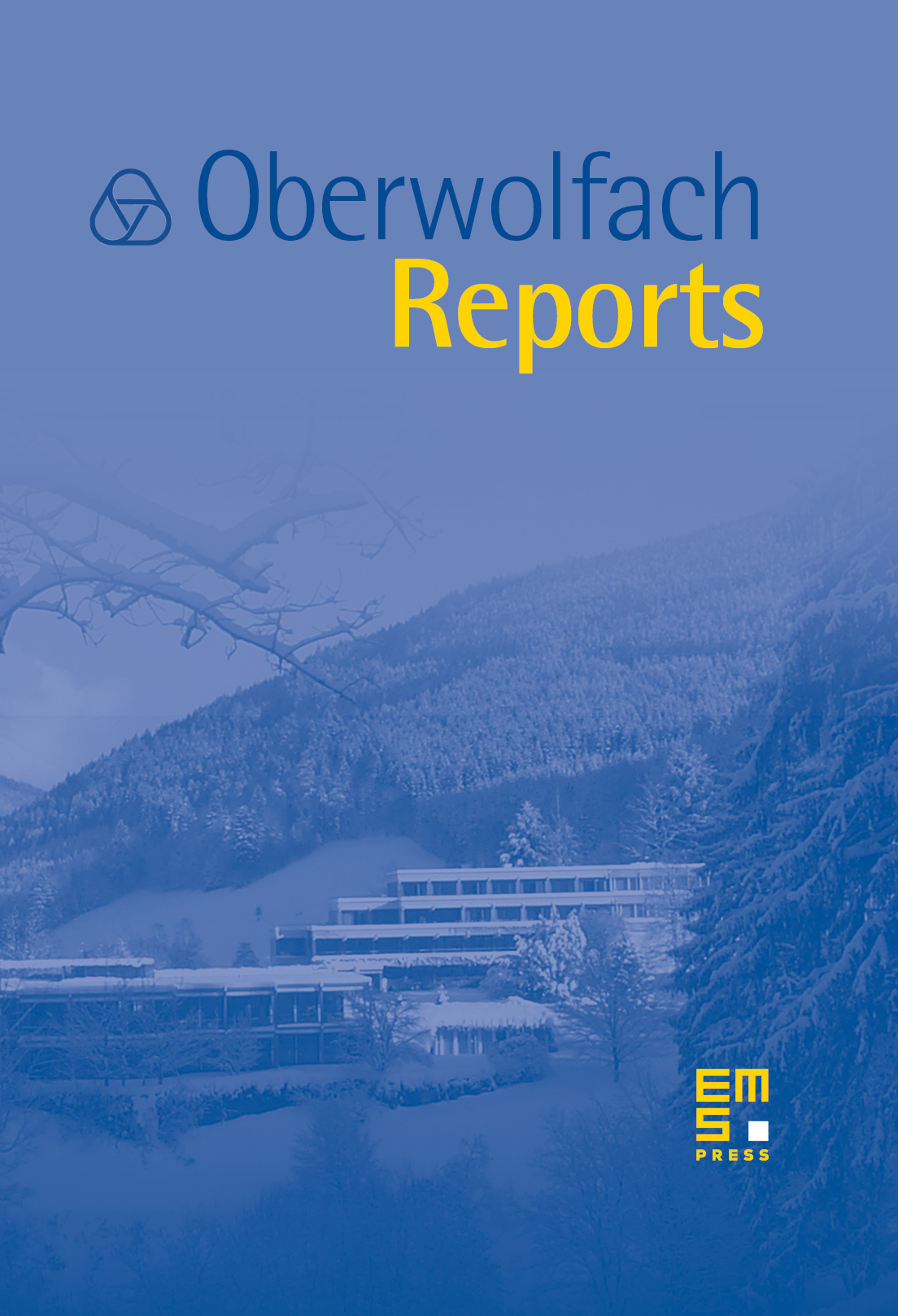
Abstract
Rapid progress in machine learning is enabling scientific advances across a range of disciplines. However, the utility of machine learning for science remains constrained by its current inability to translate insights from data about the dynamics of a system to new scientific knowledge about why those dynamics emerge, as traditionally represented by physical modelling. Mathematics is the interface that bridges data-driven and physical models of the world and can provide a foundation for delivering such knowledge. This workshop convened researchers working across domains with a shared interest in mathematics, machine learning, and their application in the sciences, to explore how tools of mathematics can help build machine learning tools for scientific discovery.
Cite this article
Neil Lawrence, Jessica Montgomery, Bernhard Schölkopf, Machine Learning for Science: Mathematics at the Interface of Data-driven and Mechanistic Modelling. Oberwolfach Rep. 20 (2023), no. 2, pp. 1453–1484
DOI 10.4171/OWR/2023/26