Classification of bivariate measures of linear association
Wiebe R. Pestman
Universidade Federal de Santa Catarina, Florianopolis, Brazil
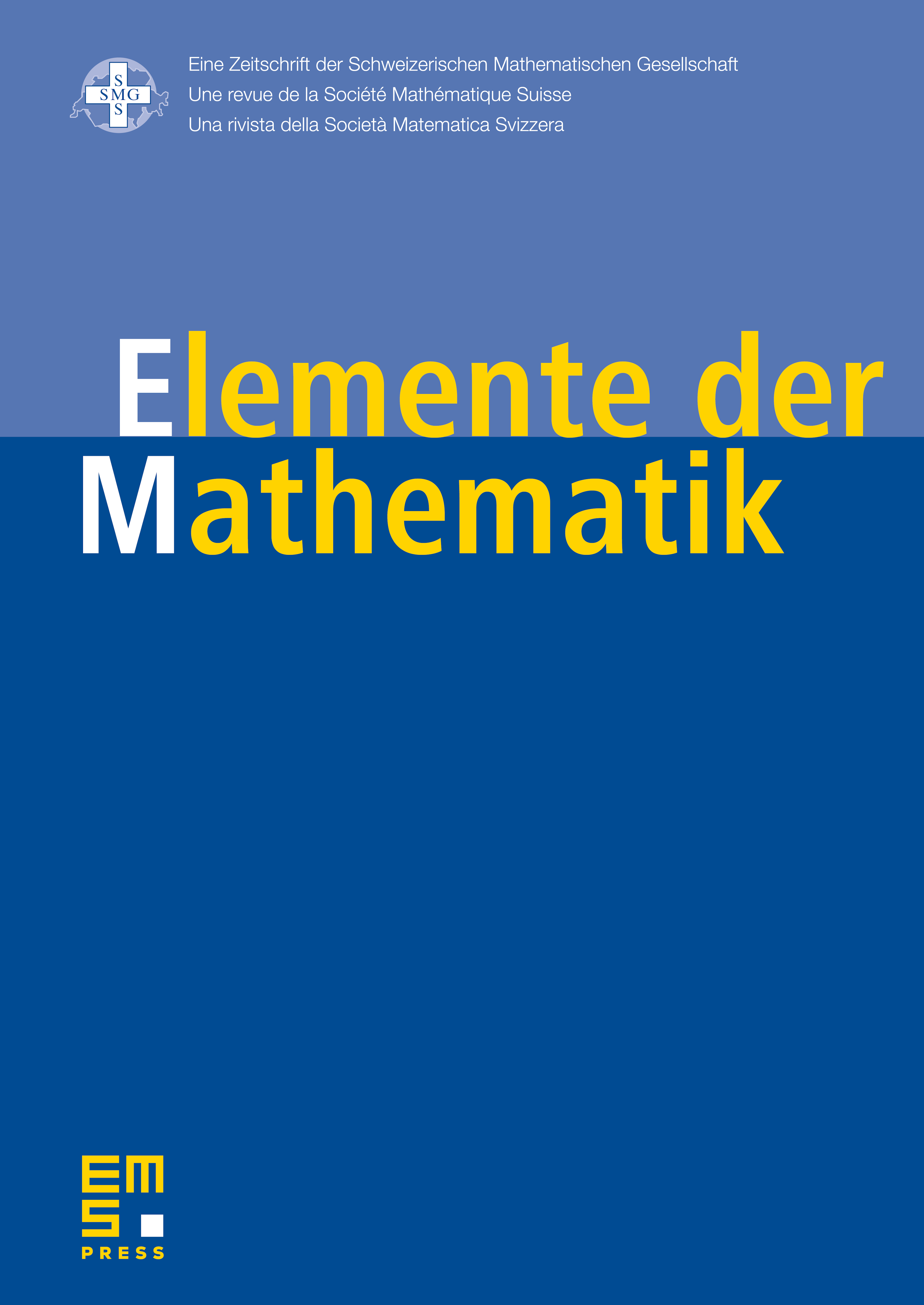
Abstract
Given an n × 2 matrix, its rows can be geometrically interpreted as a cloud of points in the plane. Such a cloud of points could show clustering around a straight line. In statistics Pearson’s (empiric) correlation coefficient is often used to capture the degree to which this phenomenon occurs in a number. This seems a peculiar usage, for Pearson’s correlation coefficient changes when the cloud of points is rotated. Hence, whereas the shape of the cloud of points is completely preserved, the very number that is supposed to characterize clustering around a line changes its values (see also [4], [5], [6]). Other measures of linear association that do better in this respect can easily be created. Such measures, however, fail as a rule to be invariant under the action of rescaling the data. It will be explained that this is no coincidence.
Cite this article
Wiebe R. Pestman, Classification of bivariate measures of linear association. Elem. Math. 65 (2010), no. 1, pp. 1–11
DOI 10.4171/EM/132