High-dimensional statistics, with applications to genome-wide association studies
Peter Bühlmann
ETH Zürich,Switzerland
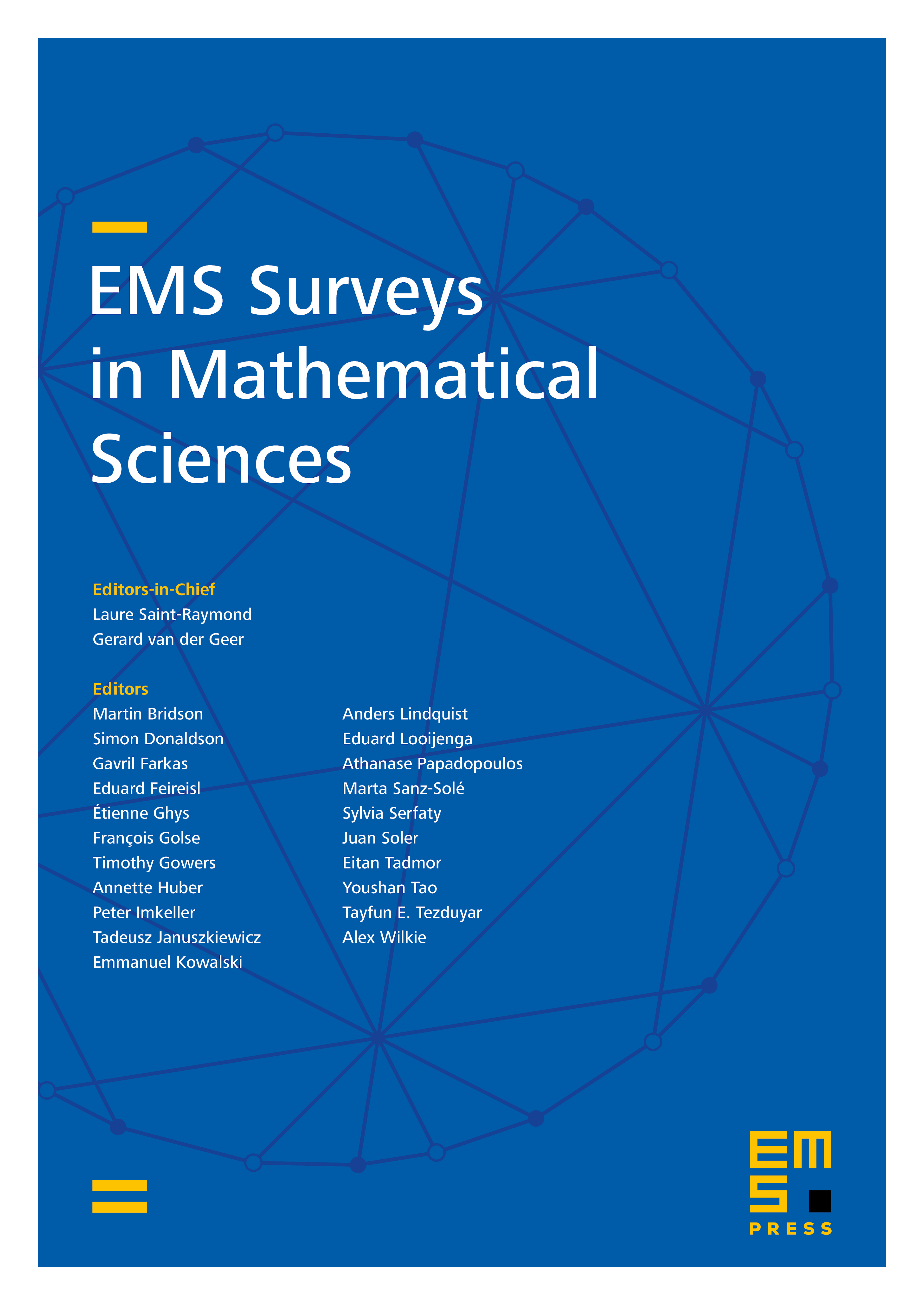
Abstract
We present a selective review on high-dimensional statistics where the dimensionality of the unknown parameter in a model can be much larger than the sample size in a dataset (e.g. the number of people in a study). Particular attention is given to recent developments for quantifying uncertainty in high-dimensional scenarios. Assessing statistical uncertainties enables to describe some degree of replicability of scientific findings, an ingredient of key importance for many applications. We also show here how modern high-dimensional statistics offers new perspectives in an important area in genetics: novel ways of analyzing genome-wide association studies, towards inferring more causal-oriented conclusions.
Cite this article
Peter Bühlmann, High-dimensional statistics, with applications to genome-wide association studies. EMS Surv. Math. Sci. 4 (2017), no. 1, pp. 45–75
DOI 10.4171/EMSS/4-1-3