Improved regret for zeroth-order adversarial bandit convex optimisation
Tor Lattimore
Google UK, London, UK
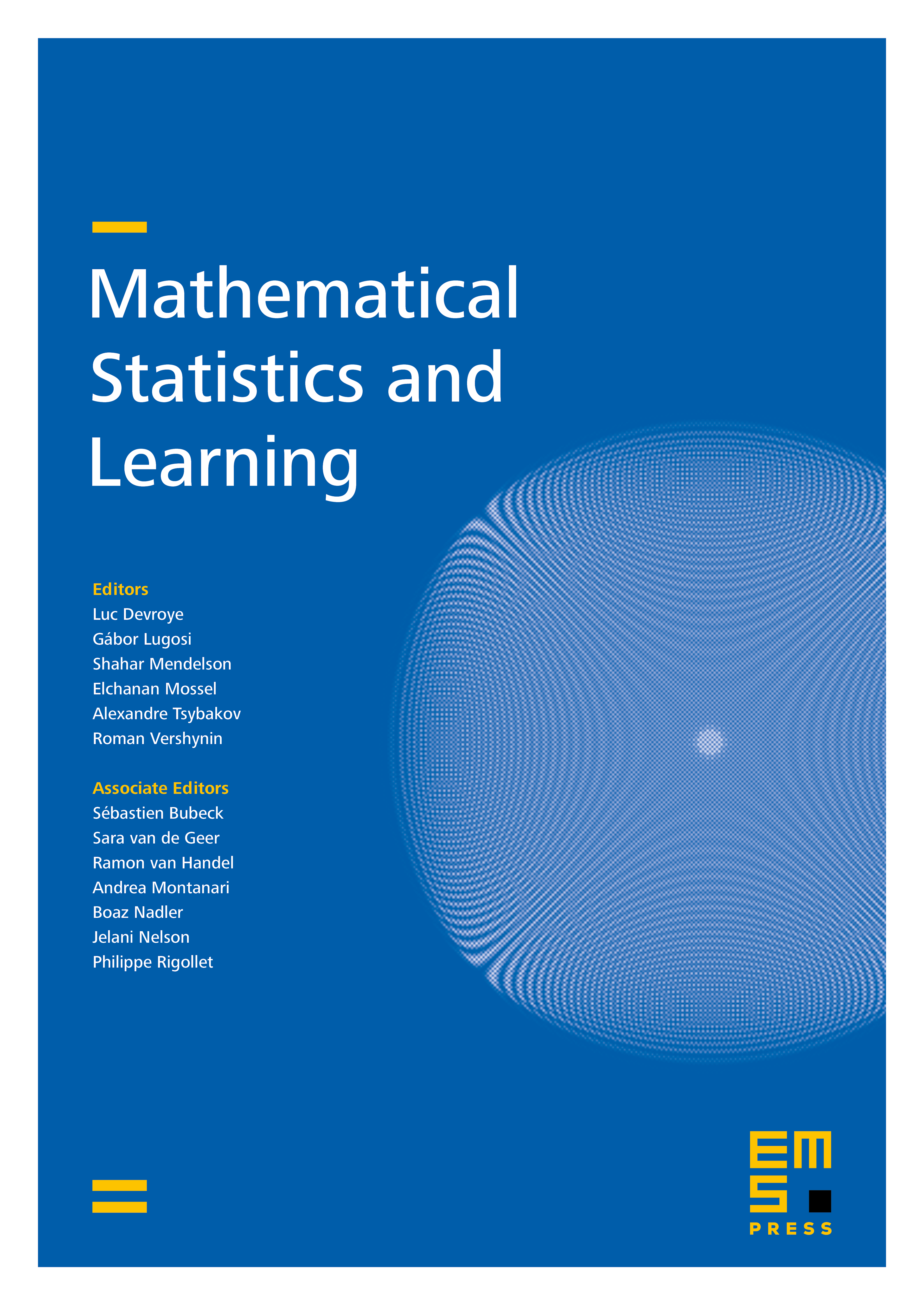
Abstract
We prove that the information-theoretic upper bound on the minimax regret for zeroth-order adversarial bandit convex optimisation is at most , where is the dimension and is the number of interactions. This improves on the bound of by Bubeck et al. (2017). The proof is based on identifying an improved exploratory distribution for convex functions.
Cite this article
Tor Lattimore, Improved regret for zeroth-order adversarial bandit convex optimisation. Math. Stat. Learn. 2 (2019), no. 3/4, pp. 311–334
DOI 10.4171/MSL/17