Compressive statistical learning with random feature moments
Rémi Gribonval
Université de Lyon, FranceGilles Blanchard
Université Paris-Saclay, Orsay, FranceNicolas Keriven
CNRS, GIPSA-lab, UMR 5216, Saint-Martin-d’Hères, FranceYann Traonmilin
CNRS, Université de Bordeaux, Talence, France
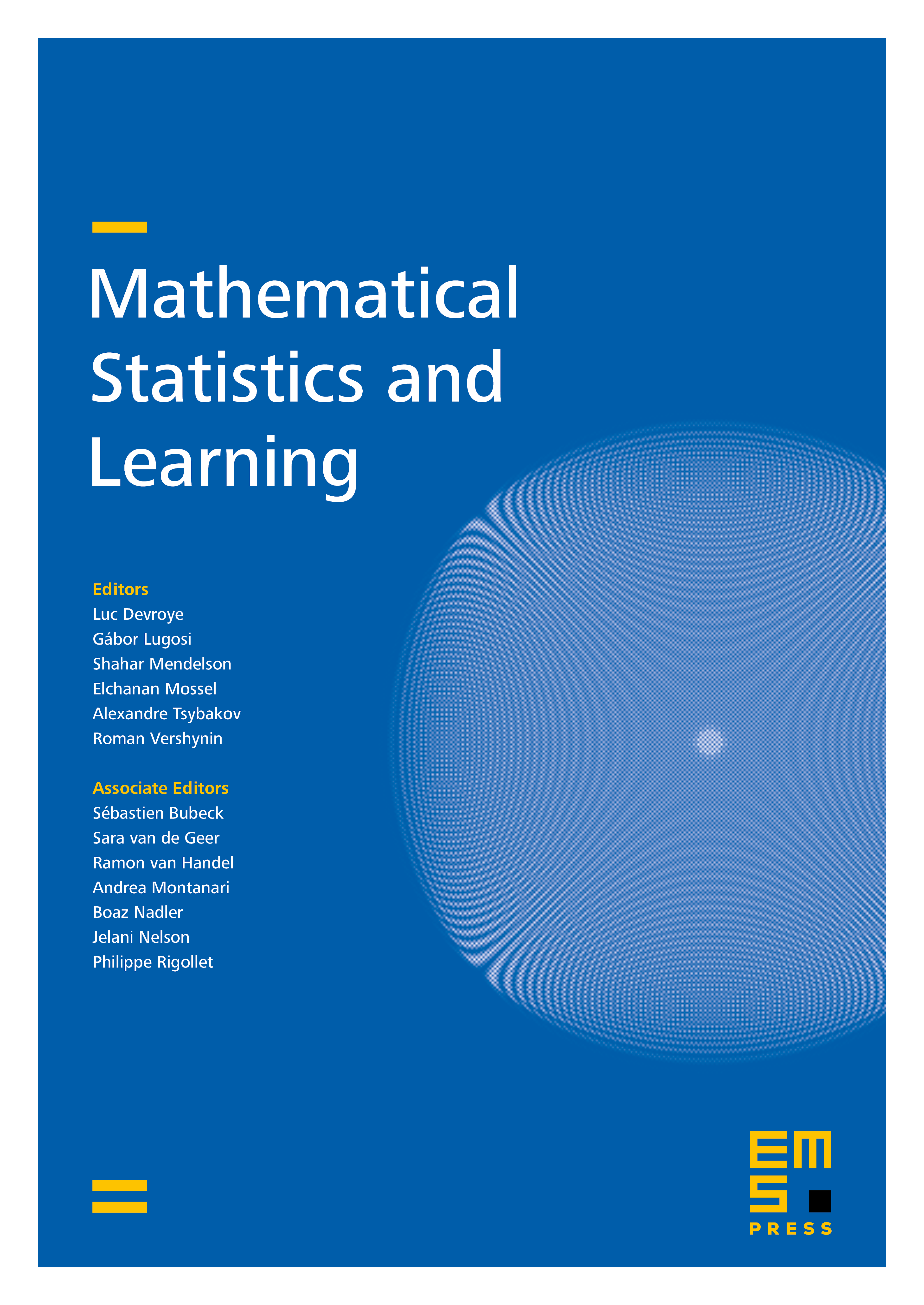
Abstract
We describe a general framework — — for resource-efficient large-scale learning: the training collection is compressed in one pass into a low-dimensional (a vector of random empirical generalized moments) that captures the information relevant to the considered learning task. A near-minimizer of the risk is computed from the sketch through the solution of a nonlinear least squares problem. We investigate sufficient sketch sizes to control the generalization error of this procedure. The framework is illustrated on compressive PCA, compressive clustering, and compressive Gaussian mixture Modeling with fixed known variance. The latter two are further developed in a companion paper.
Cite this article
Rémi Gribonval, Gilles Blanchard, Nicolas Keriven, Yann Traonmilin, Compressive statistical learning with random feature moments. Math. Stat. Learn. 3 (2020), no. 2, pp. 113–164
DOI 10.4171/MSL/20