Mini-Workshop: Frontiers in Quantile Regression
Victor Chernozhukov
Massachusetts Institute of Technology, Cambridge, USAHolger Dette
Ruhr-Universität Bochum, GermanyXuming He
University of Michigan, Ann Arbor, USARoger W. Koenker
University of Illinois at Urbana-Champaign, USA
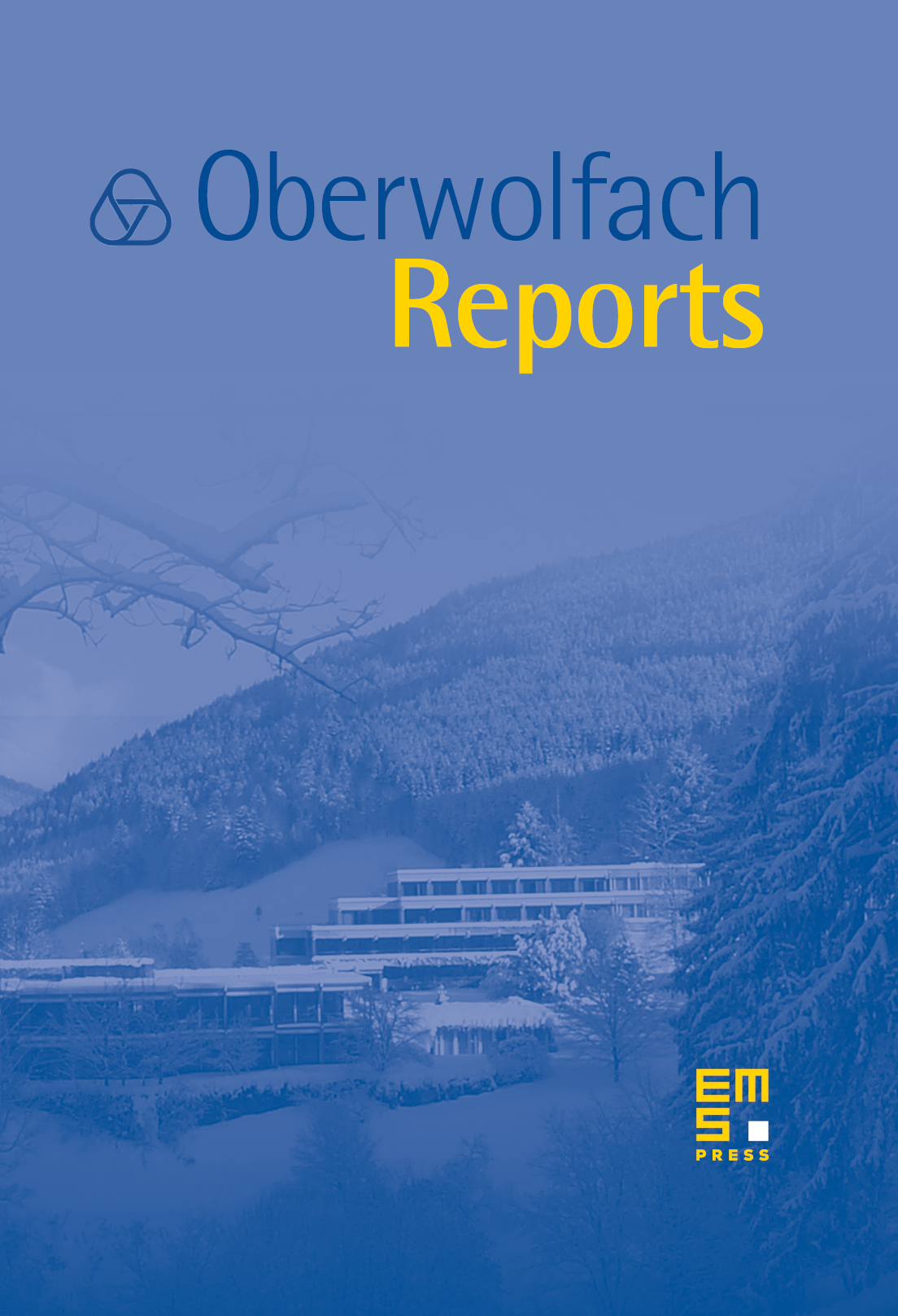
Abstract
Quantiles play an essential role in modern statistics, as emphasized by the fundamental work of Parzen (1978) and Tukey (1977). Quantile regression was introduced by Koenker and Bassett (1978) as a complement to least squares estimation (LSE) or maximum likelihood estimation (MLE) and leads to far-reaching extensions of ”classical” regression analysis by estimating families of conditional quantile surfaces, which describe the relation between a one-dimensional response y and a high dimensional predictor x. Since its introduction quantile regression has found great attraction in mathematical and applied statistics because of its natural interpretability and robustness, which yields attractive applications in such important areas as medicine, economics, engineering and environmental modeling. Although classical quantile regression theory is very well developed, the implicit definition of quantile regression still yields many new mathematical challenges such as multivariate, censored and longitudinal data, which were discussed during the workshop.
Cite this article
Victor Chernozhukov, Holger Dette, Xuming He, Roger W. Koenker, Mini-Workshop: Frontiers in Quantile Regression. Oberwolfach Rep. 9 (2012), no. 4, pp. 3339–3373
DOI 10.4171/OWR/2012/56