Mini-Workshop: Interpolation and Over-parameterization in Statistics and Machine Learning
Mihkail Belkin
University of California, San Diego, La Jolla, USAAlexandre B. Tsybakov
Institut Polytechnique de Paris, Palaiseau Cedex, FranceFanny Yang
ETH Zürich, Zürich, Switzerland
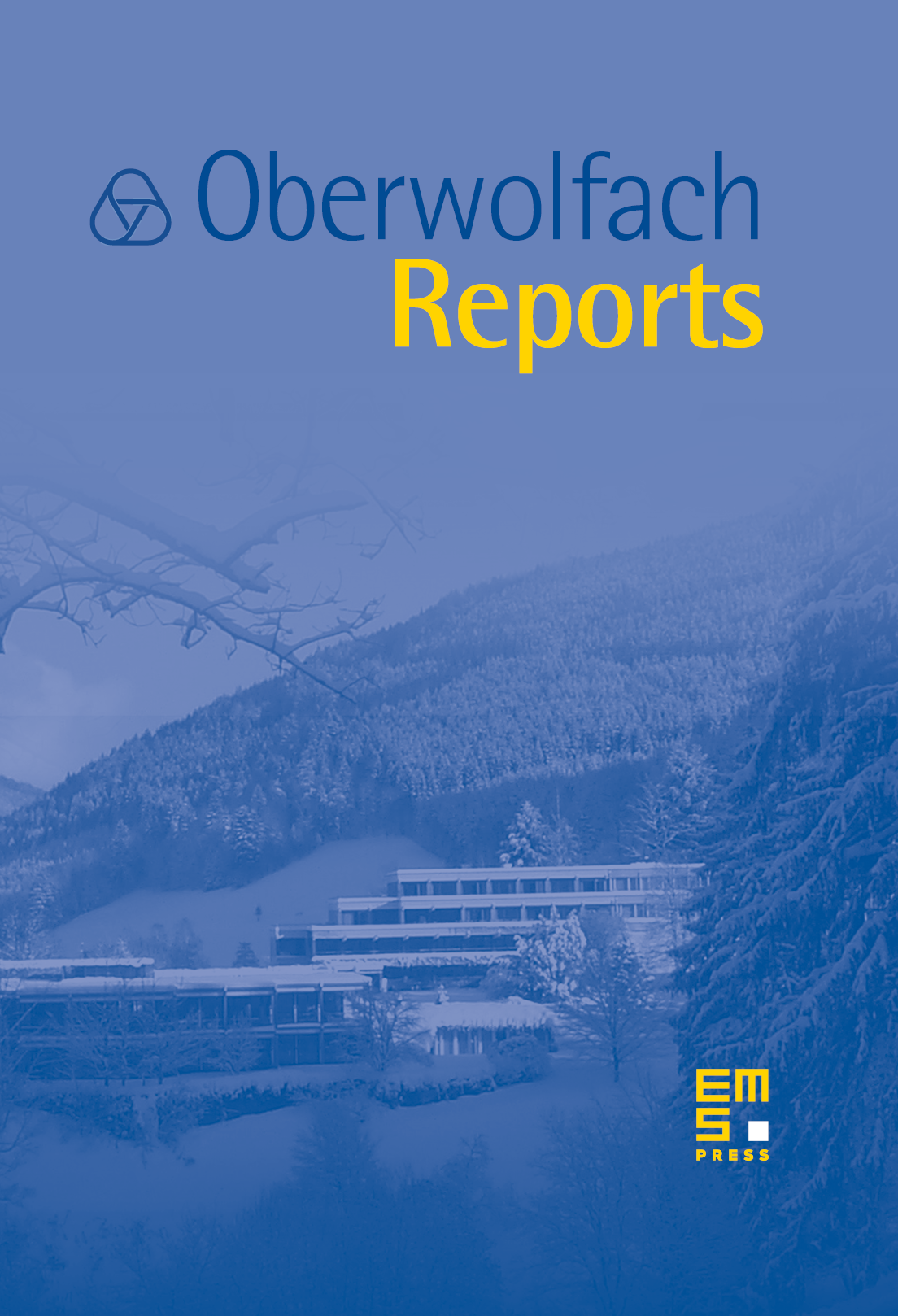
Abstract
In recent years it has become clear that, contrary to traditional statistical beliefs, methods that interpolate (fit exactly) the noisy training data, can still be statistically optimal. In particular, this phenomenon of “be- nign overfitting” or “harmless interpolation” seems to be close to the practical regimes of modern deep learning systems, and, arguably, underlies many of their behaviors. This workshop brought together experts on the emerging theory of interpolation in statistical methods, its theoretical foundations and applications to machine learning and deep learning.
Cite this article
Mihkail Belkin, Alexandre B. Tsybakov, Fanny Yang, Mini-Workshop: Interpolation and Over-parameterization in Statistics and Machine Learning. Oberwolfach Rep. 20 (2023), no. 3, pp. 2359–2376
DOI 10.4171/OWR/2023/41