Mini-Workshop: Nonlinear Approximation of High-dimensional Functions in Scientific Computing
Mathias Oster
RWTH Aachen University, GermanyJanina Schütte
Weierstraß-Institut für Angewandte Analysis und Stochastik, Berlin, GermanyPhilipp Trunschke
Université de Nantes, France
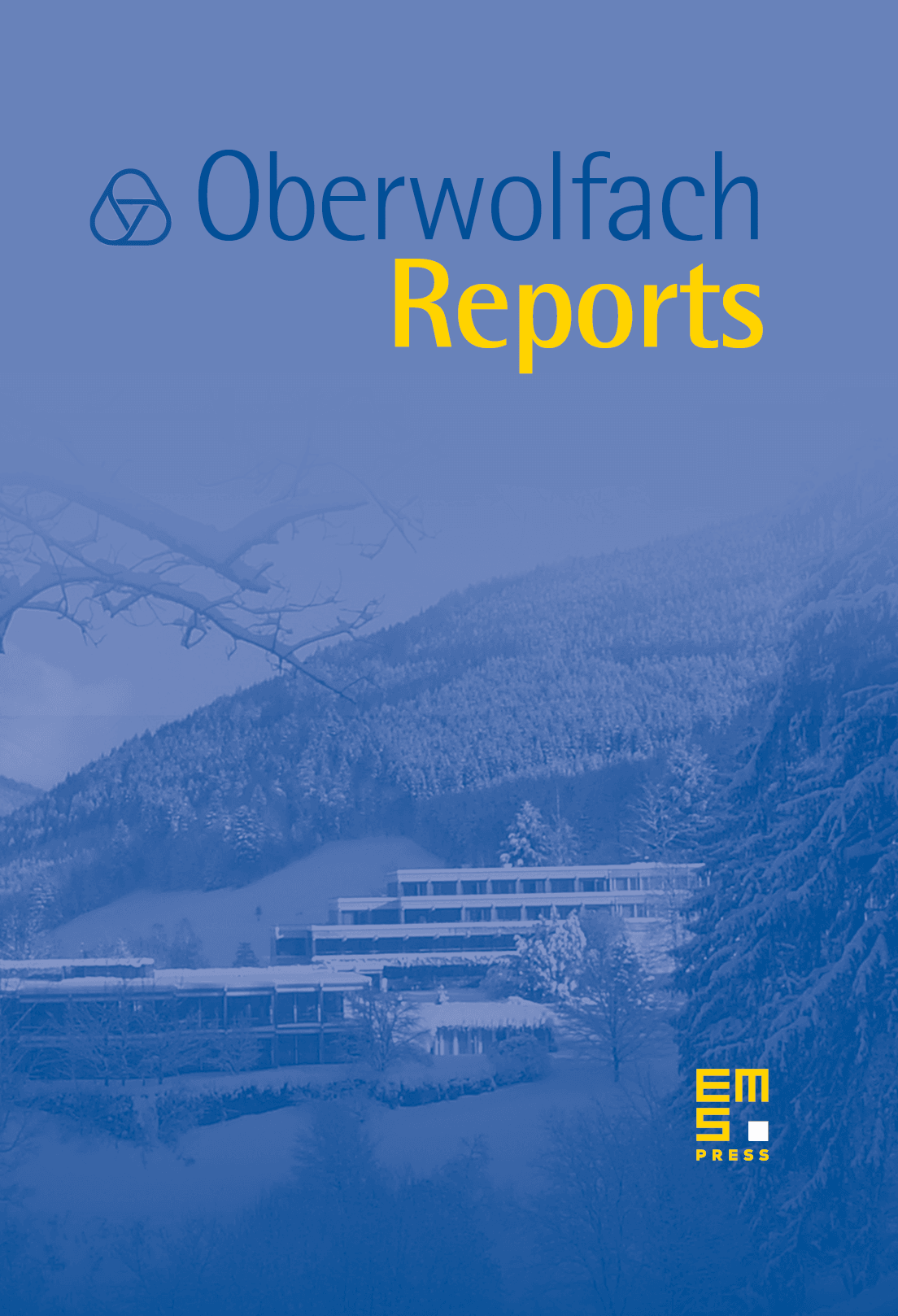
Abstract
Approximation techniques for high dimensional PDEs are crucial for contemporary scientific computing tasks and gained momentum in recent years due to the renewed interest in neural networks. It seems that especially nonlinear parametrizations will play an essential role in efficient and tractable approximations of high dimensional problems. We held a mini-workshop on the relation and possible synergy of neural networks and tensor product approximation. To reliably evaluate the prospect of different numerical experiments, the traditional talks were accompanied by live coding sessions.
Cite this article
Mathias Oster, Janina Schütte, Philipp Trunschke, Mini-Workshop: Nonlinear Approximation of High-dimensional Functions in Scientific Computing. Oberwolfach Rep. 20 (2023), no. 4, pp. 2771–2808
DOI 10.4171/OWR/2023/48