Game-theoretic Statistical Inference: Optional Sampling, Universal Inference, and Multiple Testing Based on E-values
Peter Grünwald
Centrum Wiskunde & Informatica, Amsterdam, NetherlandsAaditya Ramdas
Carnegie Mellon University, Pittsburgh, USARuodu Wang
University of Waterloo, Waterloo, CanadaJohanna Ziegel
ETH Zürich, Zürich, Switzerland
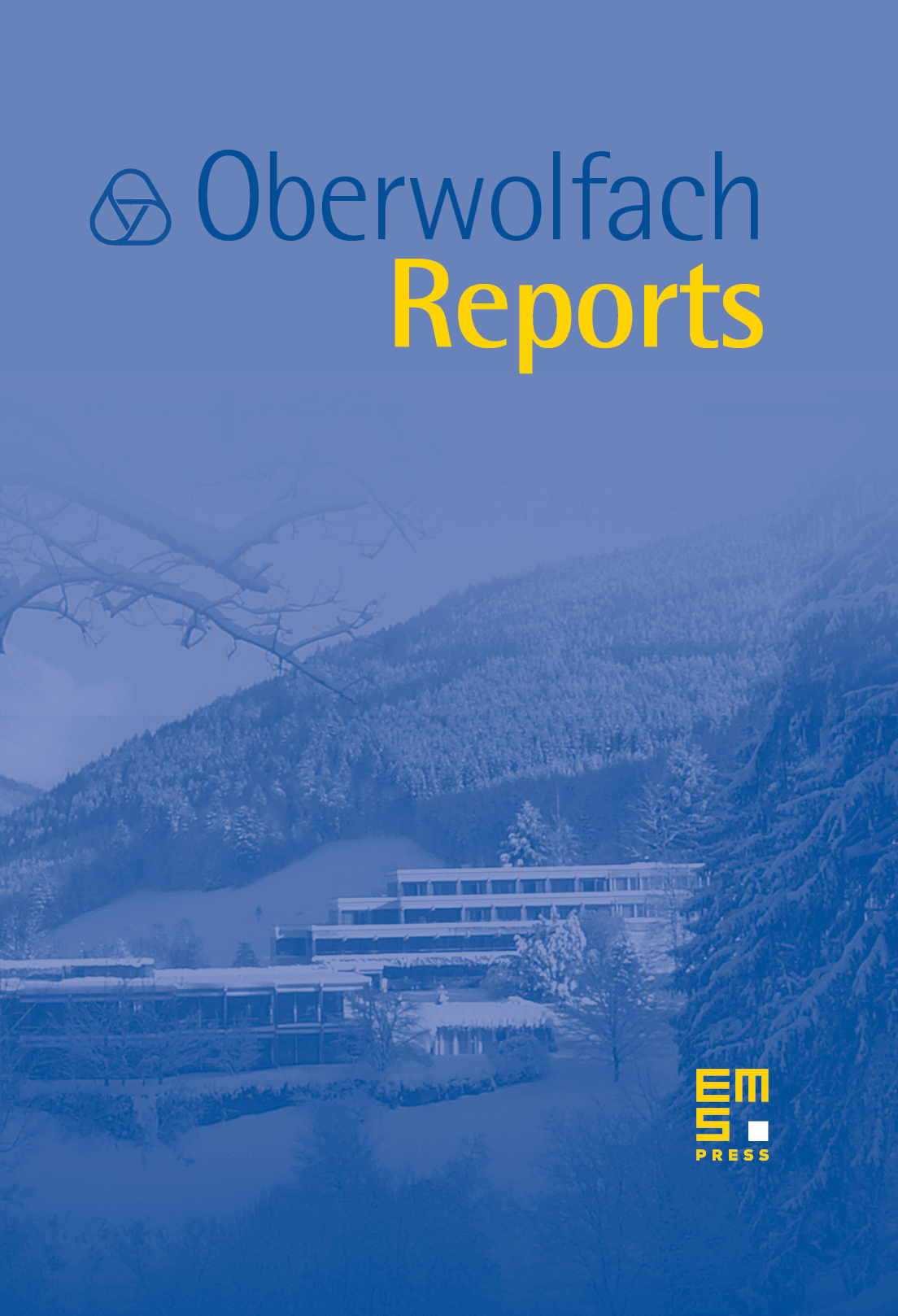
Abstract
This half-size MFO workshop brings together researchers in mathematical statistics, probability theory, machine learning, medical sciences, and economics to discuss recent developments in sequential inference. New sequential inference methods that build on nonnegative martingale techniques allow us to elegantly solve prominent shortcomings of traditional statistical hypothesis tests. Instead of p-values, they are based on e-values which have the added benefit that their meaning is much easier to communicate to applied researchers, due to their intuitive interpretation in terms of the wealth of a gambler playing a hypothetically fair game. Significant new contributions to this fast growing research area will be presented in order to stimulate collaborations, discuss and unify notation and concepts in the fields, and tackle a variety of open problems and address current major challenges.
Cite this article
Peter Grünwald, Aaditya Ramdas, Ruodu Wang, Johanna Ziegel, Game-theoretic Statistical Inference: Optional Sampling, Universal Inference, and Multiple Testing Based on E-values. Oberwolfach Rep. 21 (2024), no. 2, pp. 1339–1386
DOI 10.4171/OWR/2024/24