Mathematical and Algorithmic Aspects of Data Assimilation in the Geosciences
Andreas Griewank
Humboldt-Universität zu Berlin, GermanySebastian Reich
Universität Potsdam, GermanyIan Roulstone
University of Surrey, Guildford, UKAndrew M. Stuart
University of Warwick, Coventry, UK
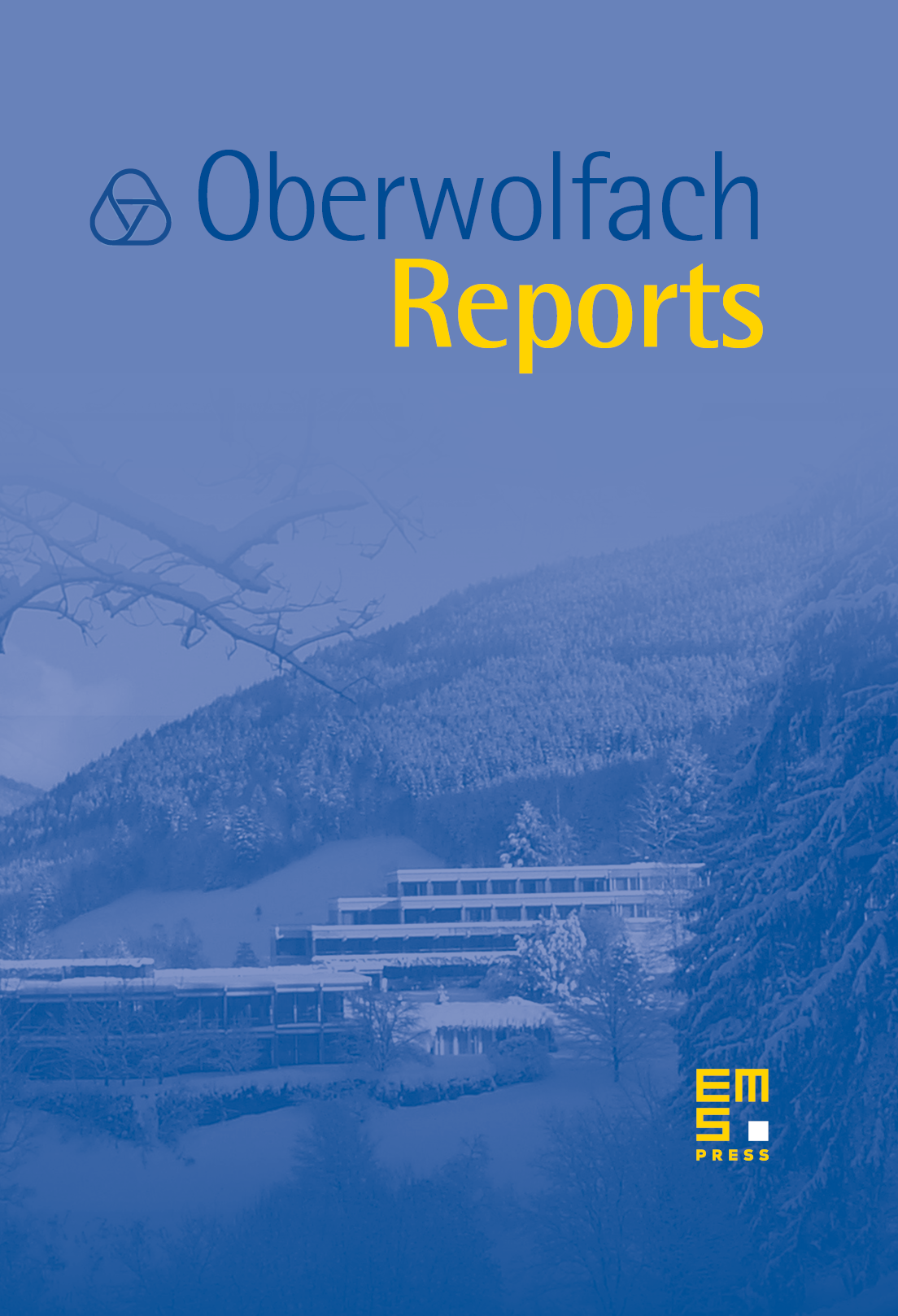
Abstract
The field of “Data Assimilation” has been driven by applications from the geosciences where complex mathematical models are interfaced with observational data in order to improve model forecasts. Mathematically, data assimilation is closely related to filtering and smoothing on the one hand and inverse problems and statistical inference on the other. Key challenges of data assimilation arise from the high-dimensionality of the underlying models, combined with systematic spatio-temporal model errors, pure model uncertainty quantification and relatively sparse observation networks. Advances in the field of data assimilation will require combination of a broad range of mathematical techniques from differential equations, statistics, machine learning, probability, scientific computing and mathematical modeling, together with insights from practitioners in the field. The workshop brought together a collection of scientists representing this broad spectrum of research strands.
Cite this article
Andreas Griewank, Sebastian Reich, Ian Roulstone, Andrew M. Stuart, Mathematical and Algorithmic Aspects of Data Assimilation in the Geosciences. Oberwolfach Rep. 13 (2016), no. 4, pp. 2705–2748
DOI 10.4171/OWR/2016/47