Statistics for Data with Geometric Structure
Aasa Feragen
University of Copenhagen, DenmarkThomas Hotz
Technische Universität Ilmenau, GermanyStephan Huckemann
Georg-August-Universität Göttingen, GermanyEzra Miller
Duke University, Durham, USA
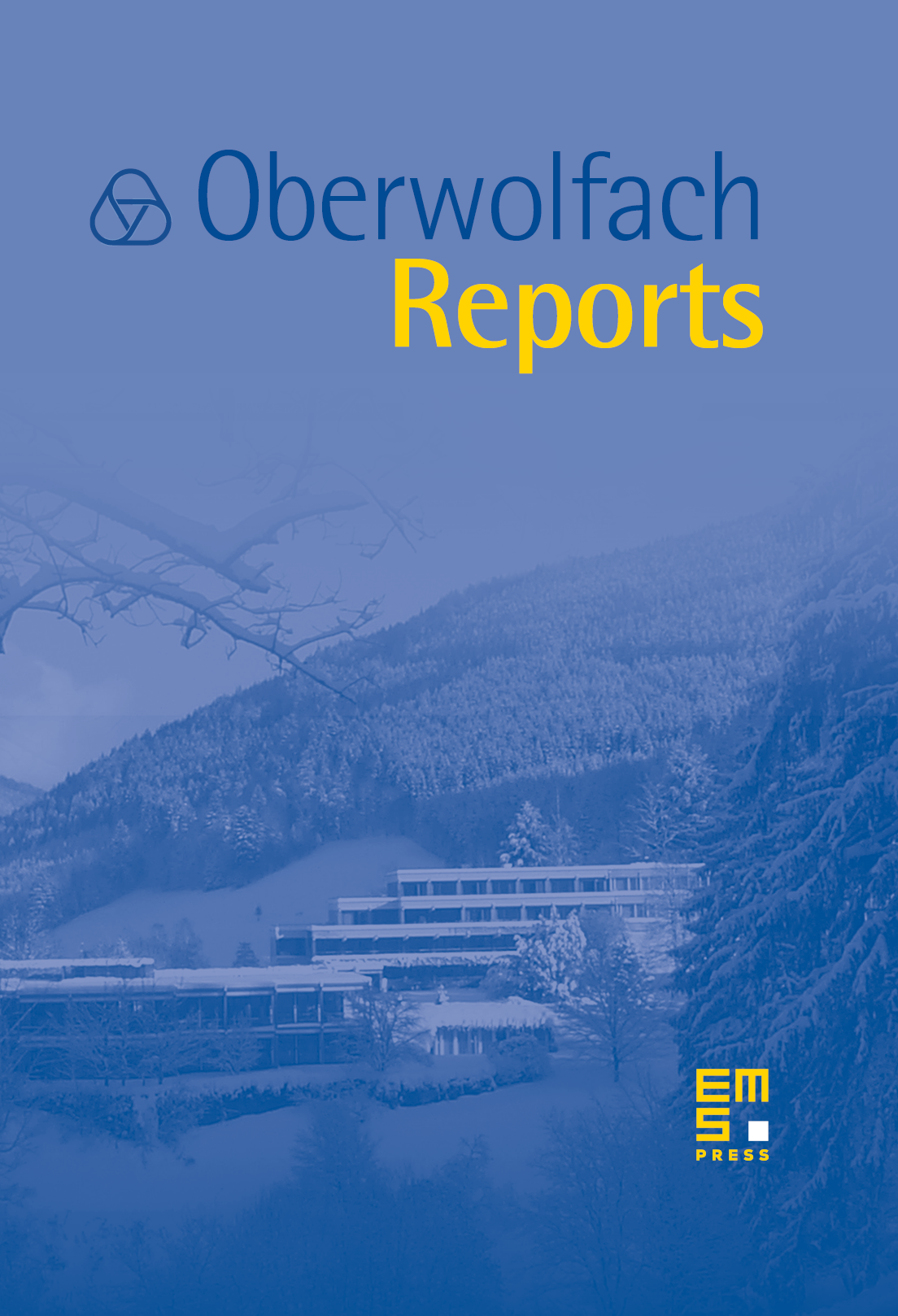
Abstract
Statistics for data with geometric structure is an active and diverse topic of research. Applications include manifold spaces in directional data or symmetric positive definite matrices and some shape representations. But in some cases, more involved metric spaces like stratified spaces play a crucial role in different ways. On the one hand, phylogenetic trees are represented as points in a stratified data space, whereas branching trees, for example of veins, are data objects, whose stratified structure is of essentia importance. For the latter case, one important tool is persistent homology, which is currently a very active area of research. As data sets become not only larger but also more complex, the need for theoretical and methodological progress in dealing with data on non-Euclidean spaces or data objects with nontrivial geometric structure is growing. A number of fundamental results have been achieved recently and the development of new methods for refined, more informative data representation is ongoing. Two complimentary approaches are pursued: on the one hand developing sophisticated new parameters to describe the data, like persistent homology, and on the other hand achieving simpler representations in terms of given parameters, like dimension reduction. Some foundational works in stochastic process theory on manifolds open the doors to this field and stochastic analysis on manifolds, thus enabling a well-founded treatment of non-Euclidean dynamic data. The results presented in the workshop by leading experts in the field are great accomplishments of collaboration between mathematicians from statistics, geometry and topology and the open problems which were discussed show the need for an expansion of this interdisciplinary effort, which could also tie in more closely with computer science.
Cite this article
Aasa Feragen, Thomas Hotz, Stephan Huckemann, Ezra Miller, Statistics for Data with Geometric Structure. Oberwolfach Rep. 15 (2018), no. 1, pp. 125–186
DOI 10.4171/OWR/2018/3