Statistical Methodology and Theory for Functional and Topological Data
Aurore Delaigle
The University of Melbourne, Parkville, AustraliaAlexander Meister
Universität Rostock, GermanyVictor M. Panaretos
Ecole Polytechnique Fédérale de Lausanne, SwitzerlandLarry Wasserman
Carnegie Mellon University, Pittsburgh, USA
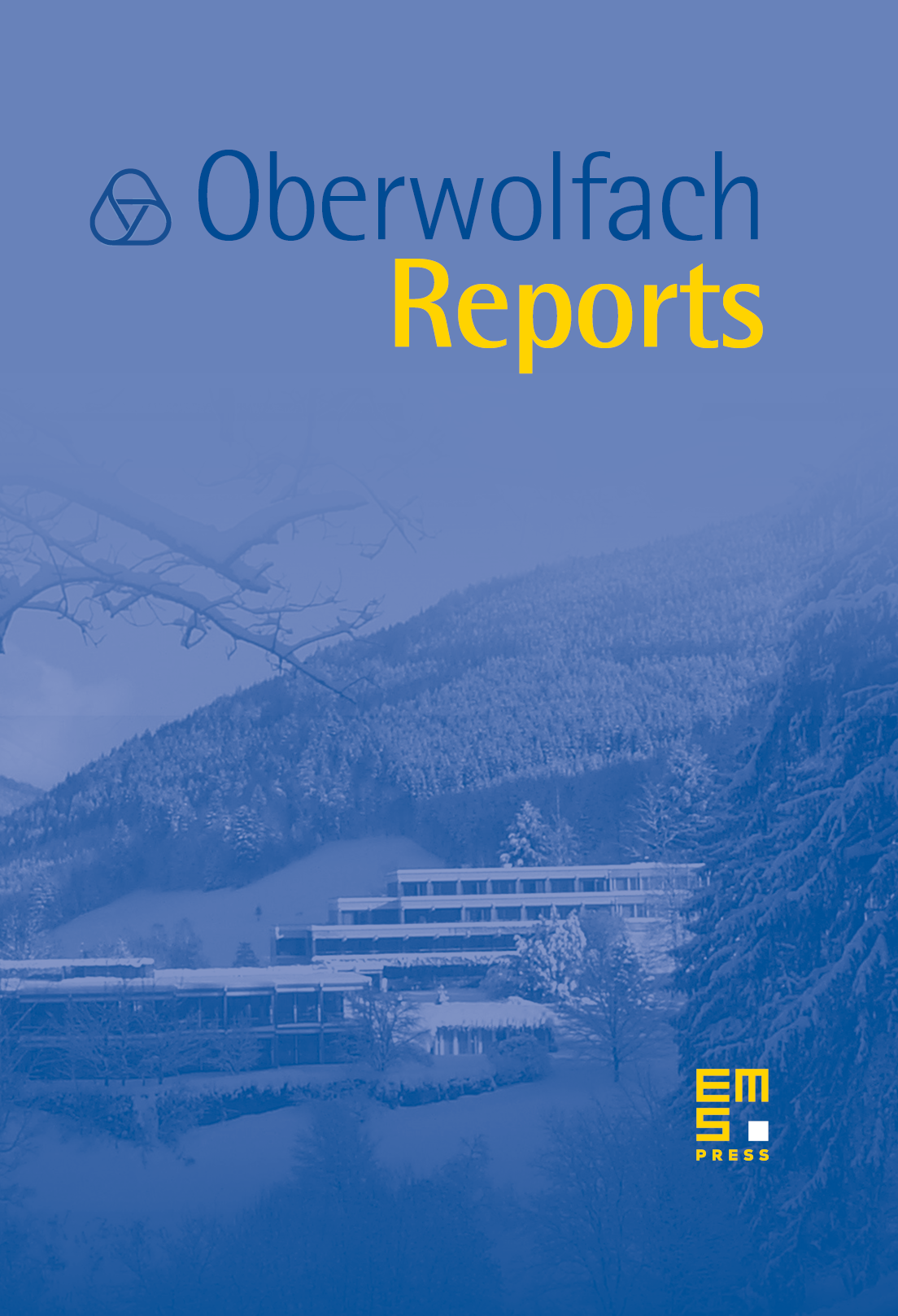
Abstract
The workshop focuses on the statistical analysis of complex data which cannot be represented as realizations of finite-dimensional random vectors. An example of such data are functional data. They arise in a variety of climate, biological, medical, physical and engineering problems, where the observations can be represented by curves and surfaces. Fast advances in technology continuously produce a deluge of bigger data with even more complex structures such as arteries in the brain, bones of a human body or social networks. This has sparked enormous interest in more general statistical problems where the random observations are elements of abstract topological spaces.
The workshop will stimulate development of new statistical methods for these types of data and will be an ideal platform for discussing their theoretical properties (e.g. asymptotic optimality), computational performance, and new exciting applications in areas such as machine learning, image analysis, biometrics and econometrics.
Cite this article
Aurore Delaigle, Alexander Meister, Victor M. Panaretos, Larry Wasserman, Statistical Methodology and Theory for Functional and Topological Data. Oberwolfach Rep. 16 (2019), no. 2, pp. 1697–1735
DOI 10.4171/OWR/2019/28