Mini-Workshop: Level Sets and Depth Contours in High Dimensional Data
Mia Hubert
Katholieke Universiteit Leuven, Leuven-Heverlee, BelgiumJun Li
University of California, Riverside, USAWolfgang Polonik
University of California at Davis, USARobert J. Serfling
University of Texas at Dallas, Richardson, USA
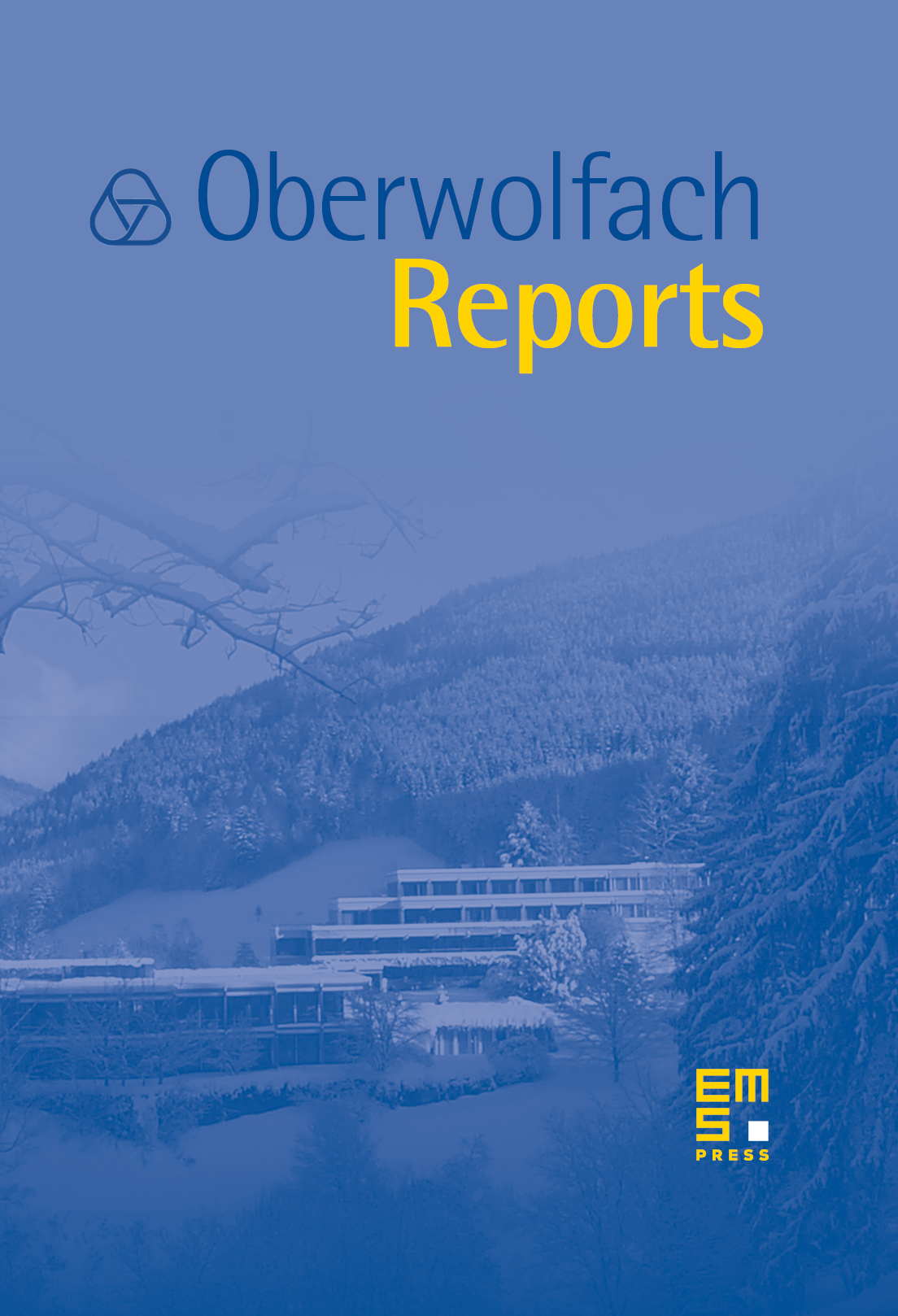
Abstract
Extraction of information about the distribution underlying a high-dimensional data set is a formidable, complex problem dominating modern nonparametric statistics. Two general strategies are (i) to extract merely qualitative information, such as modality or other shape information, and (ii) to consider relatively simple inference problems, such as binary classification. One approach toward (i) and (ii) is based on measuring qualitative information via mass concentration functions. Another approach is based on multivariate depth functions and inherently addresses issues of robustness. Having different orientations and aims, these approaches have evolved in parallel with little interaction. Yet they both in common implicitly involve level set estimation as a major tool. This mini-workshop was the first serious attempt to study and exploit such interconnections between these approaches. Researchers from both areas exchanged ideas toward forging a novel, synergistic approach that fruitfully strengthens the roles of mass concentration and depth methods in statistical inference for multivariate data. Foundations for level set estimation as a general statistical method were explored. Deeper understanding of the so-called generalized quantiles approach was pursued. Application to binary classification, a pervasive problem in modern statistics, received intensive special attention.
Cite this article
Mia Hubert, Jun Li, Wolfgang Polonik, Robert J. Serfling, Mini-Workshop: Level Sets and Depth Contours in High Dimensional Data. Oberwolfach Rep. 8 (2011), no. 1, pp. 691–717
DOI 10.4171/OWR/2011/13