Computation and Learning in High Dimensions
Albert Cohen
Sorbonne Université, Paris, FranceWolfgang Dahmen
University of South Carolina, Columbia, USARonald A. DeVore
Texas A&M University, College Station, USAAngela Kunoth
Universität zu Köln, Germany
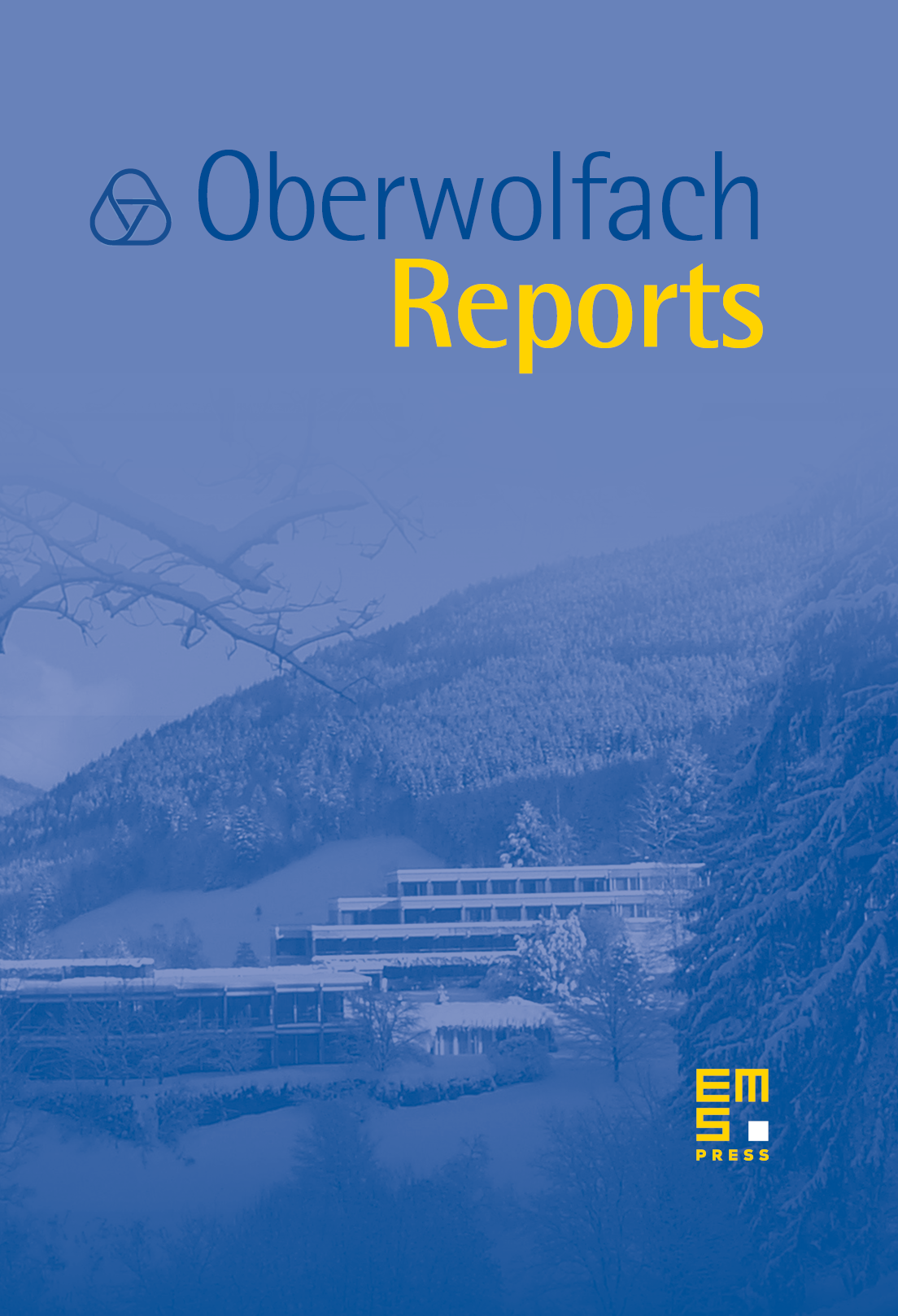
Abstract
The most challenging problems in science often involve the learning and accurate computation of high dimensional functions. High-dimensionality is a typical feature for a multitude of problems in various areas of science. The so-called curse of dimensionality typically negates the use of traditional numerical techniques for the solution of high-dimensional problems. Instead, novel theoretical and computational approaches need to be developed to make them tractable and to capture fine resolutions and relevant features. Paradoxically, increasing computational power may even serve to heighten this demand, since the wealth of new computational data itself becomes a major obstruction. Extracting essential information from complex probleminherent structures and developing rigorous models to quantify the quality of information in a high-dimensional setting pose challenging tasks from both theoretical and numerical perspective. This has led to the emergence of several new computational methodologies, accounting for the fact that by now well understood methods drawing on spatial localization and mesh-refinement are in their original form no longer viable. Common to these approaches is the nonlinearity of the solution method. For certain problem classes, these methods have drastically advanced the frontiers of computability. The most visible of these new methods is deep learning. Although the use of deep neural networks has been extremely successful in certain application areas, their mathematical understanding is far from complete.
This workshop proposed to deepen the understanding of the underlying mathematical concepts that drive this new evolution of computational methods and to promote the exchange of ideas emerging in various disciplines about how to treat multiscale and high-dimensional problems.
Cite this article
Albert Cohen, Wolfgang Dahmen, Ronald A. DeVore, Angela Kunoth, Computation and Learning in High Dimensions. Oberwolfach Rep. 18 (2021), no. 3, pp. 1915–1963
DOI 10.4171/OWR/2021/36