High dimensional statistical inference and random matrices
Iain M. Johnstone
Stanford University, USA
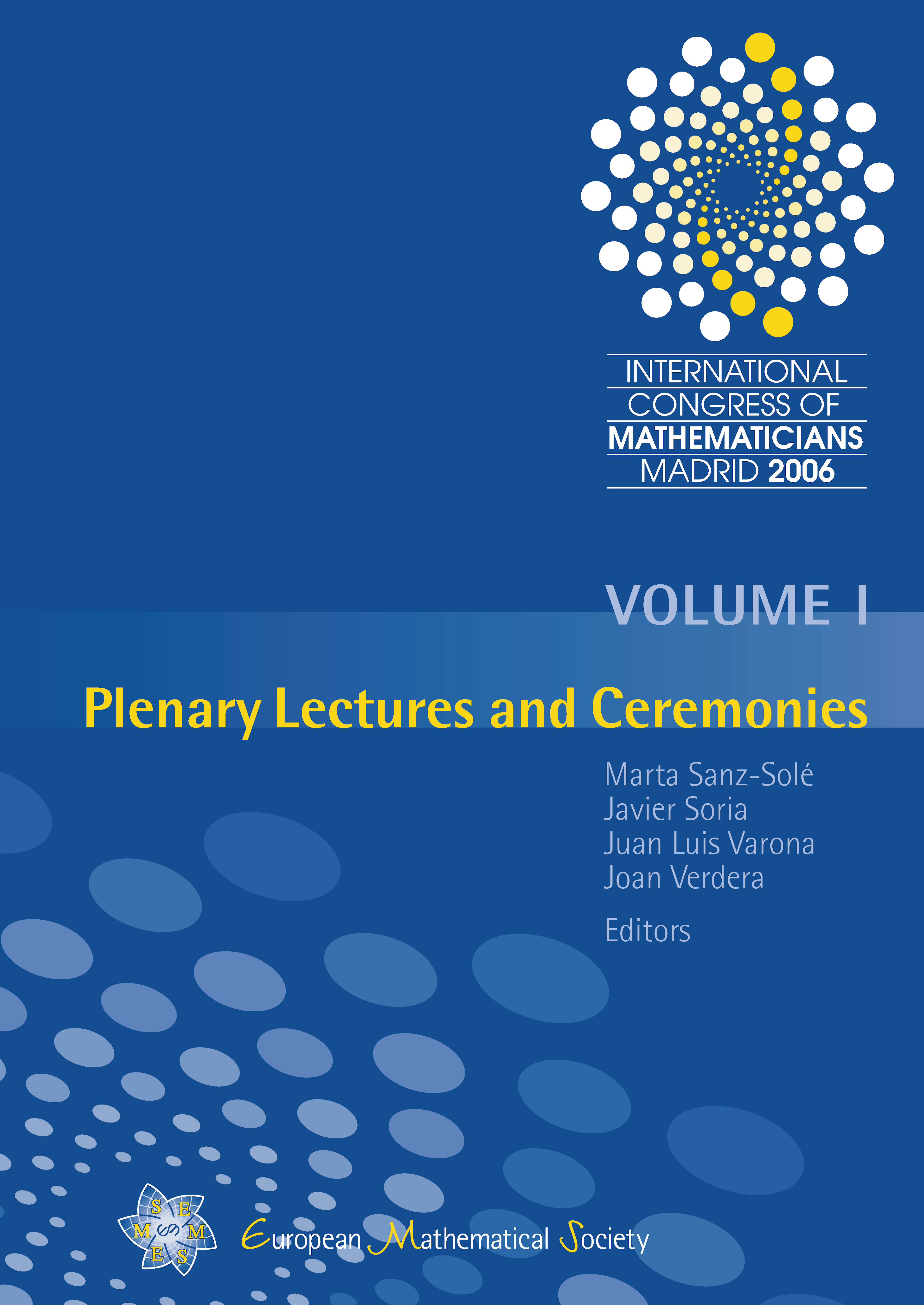
A subscription is required to access this book chapter.
Abstract
Multivariate statistical analysis is concerned with observations on several variables which are thought to possess some degree of inter-dependence. Driven by problems in genetics and the social sciences, it first flowered in the earlier half of the last century. Subsequently, random matrix theory (RMT) developed, initially within physics, and more recently widely in mathematics. While some of the central objects of study in RMT are identical to those of multivariate statistics, statistical theory was slow to exploit the connection. However, with vast data collection ever more common, data sets now often have as many or more variables than the number of individuals observed. In such contexts, the techniques and results of RMT have much to offer multivariate statistics. The paper reviews some of the progress to date.