Compressive sampling
Emmanuel J. Candès
California Institute of Technology, Pasadena, USA
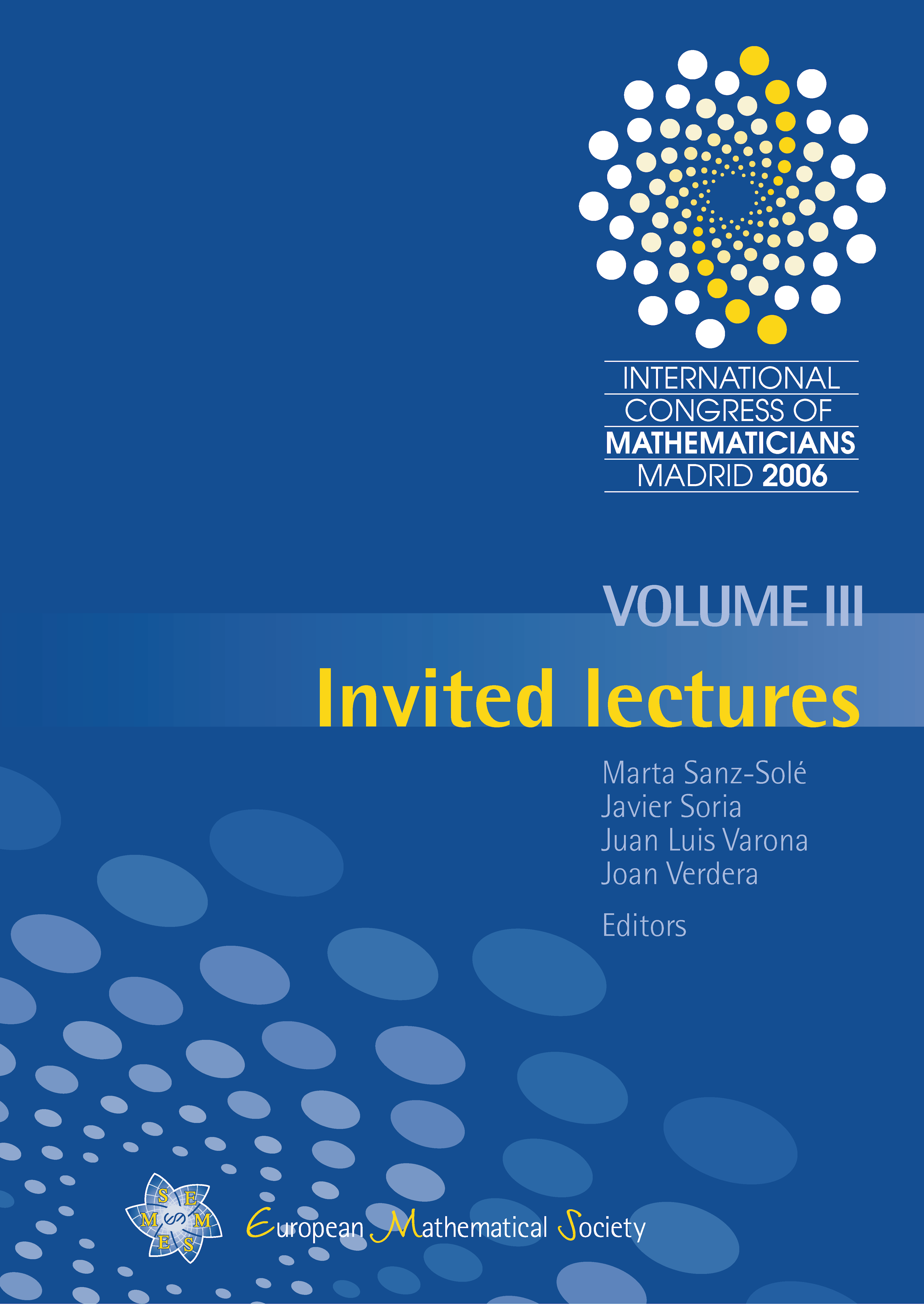
A subscription is required to access this book chapter.
Abstract
Conventional wisdom and common practice in acquisition and reconstruction of images from frequency data follow the basic principle of the Nyquist density sampling theory. This principle states that to reconstruct an image, the number of Fourier samples we need to acquire must match the desired resolution of the image, i.e. the number of pixels in the image. This paper surveys an emerging theory which goes by the name of “compressive sampling” or “compressed sensing,” and which says that this conventional wisdom is inaccurate. Perhaps surprisingly, it is possible to reconstruct images or signals of scientific interest accurately and sometimes even exactly from a number of samples which is far smaller than the desired resolution of the image/signal, e.g. the number of pixels in the image.
It is believed that compressive sampling has far reaching implications. For example, it suggests the possibility of new data acquisition protocols that translate analog information into digital form with fewer sensors than what was considered necessary. This new sampling theory may come to underlie procedures for sampling and compressing data simultaneously.
In this short survey, we provide some of the key mathematical insights underlying this new theory, and explain some of the interactions between compressive sampling and other fields such as statistics, information theory, coding theory, and theoretical computer science.