Analysis of high-dimensional distributions using pathwise methods
Ronen Eldan
Department of Mathematics, Weizmann Institute of Science, Rehovot 76100, Israel
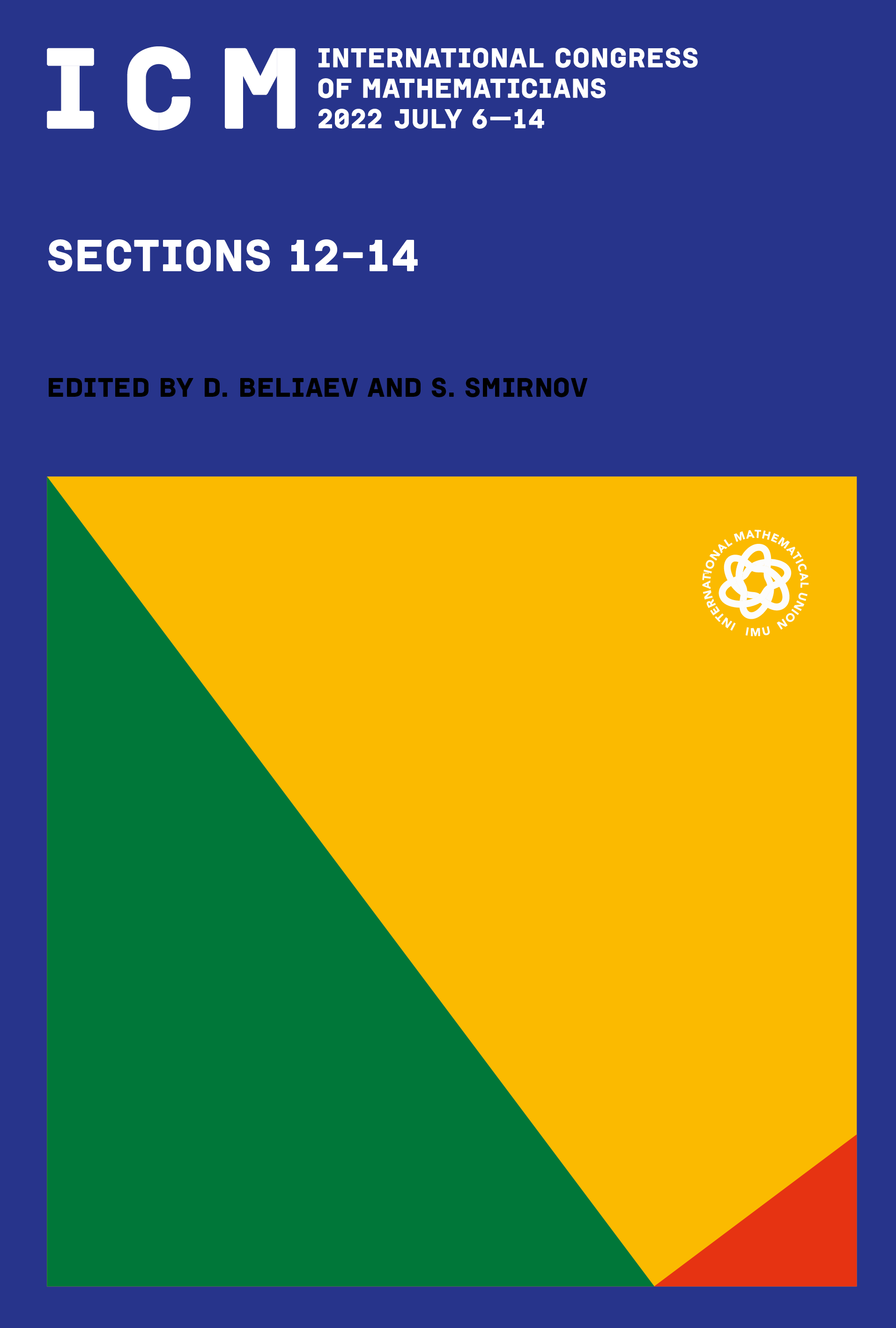
This book chapter is published open access.
Abstract
The goal of this note is to present an emerging method in the analysis of high-dimensional distributions, which exhibits applications to several mathematical fields, such as functional analysis, convex and discrete geometry, combinatorics, and mathematical physics. The method is based on pathwise analysis: One constructs a stochastic process, driven by Brownian motion, associated with the high-dimensional distribution in hand. Quantities of interest related to the distribution, such as covariance, entropy, and spectral gap, are then expressed via corresponding properties of the stochastic process, such as quadratic variation, making the former tractable through the analysis the latter. We focus on one particular manifestation of this approach, the Stochastic Localization process. We review several results which can be obtained using Stochastic Localization and outline the main steps towards their proofs. By doing so, we try to demonstrate some of the ideas and advantages of the pathwise approach. We focus on two types of results relevant to high-dimensional distributions: The first one has to do with dimension-free concentration bounds, manifested by functional inequalities which have no explicit dependence on the dimension. Our main focus in this respect will be on the Kannan–Lovász–Simonovits conjecture, concerning the isoperimetry of high-dimensional log-concave measures. Additionally, we discuss concentration inequalities for Ising models and expansion bounds for complex-analytic sets. The second type of results concern the decomposition of a high-dimensional measure into mixtures of measures attaining a simple structure, with applications to mean-field approximations.