Theory of graph neural networks: representation and learning
Stefanie Jegelka
Department of EECS, MIT, Cambridge, USA
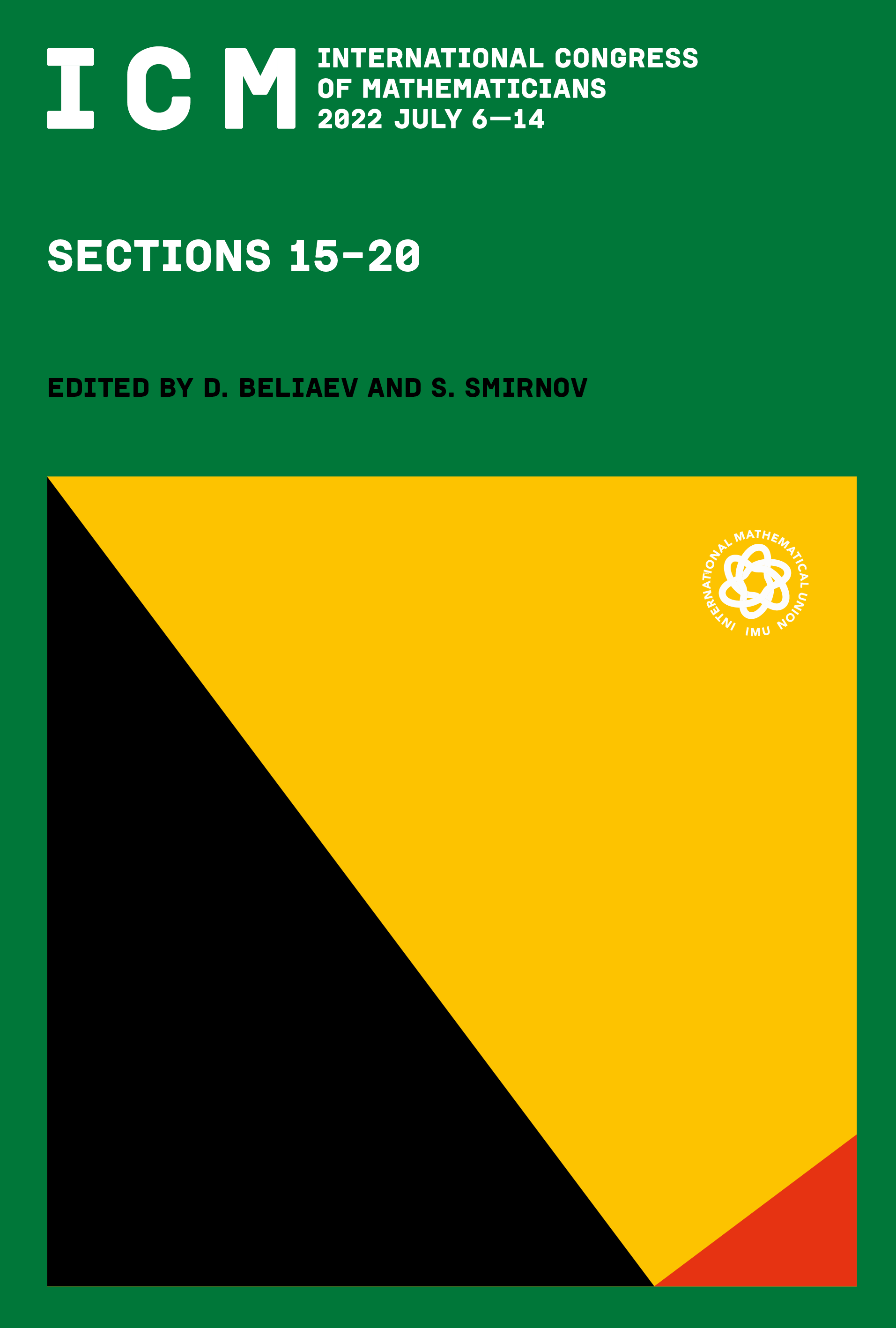
Download Chapter PDF
This book chapter is published open access.
Abstract
Graph Neural Networks (GNNs), neural network architectures targeted to learning representations of graphs, have become a popular learning model for prediction tasks on nodes, graphs and configurations of points, with wide success in practice. This article summarizes a selection of emerging theoretical results on approximation and learning properties of widely used message passing GNNs and higher-order GNNs, focusing on representation, generalization, and extrapolation. Along the way, it summarizes broad mathematical connections.