MCMC methods for sampling function space
Alexandros Beskos
University of Warwick, Coventry, UKAndrew M. Stuart
University of Warwick, Coventry, UK
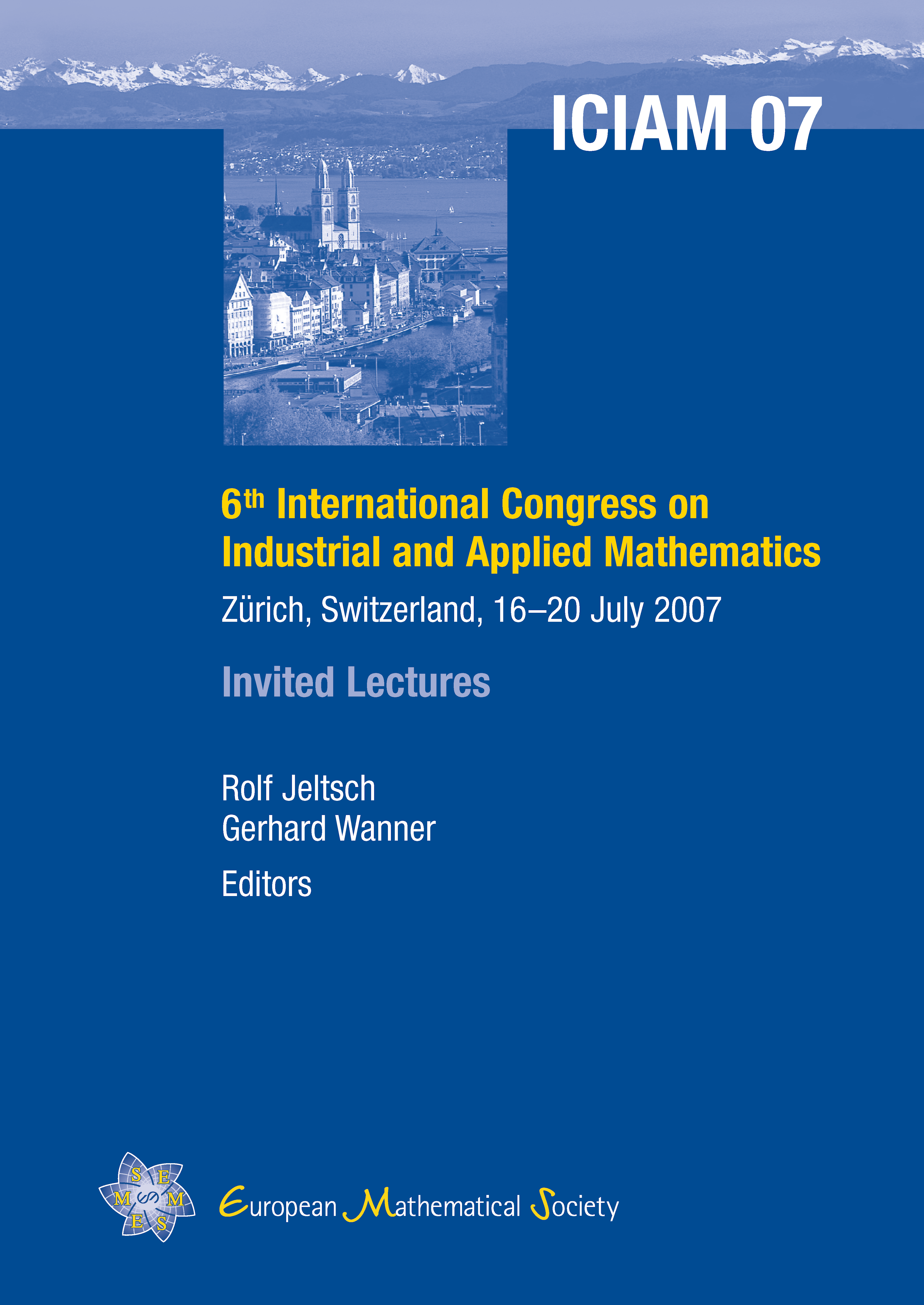
A subscription is required to access this book chapter.
Abstract
Applied mathematics is concerned with developing models with predictive capability, and with probing those models to obtain qualitative and quantitative insight into the phenomena being modelled. Statistics is data-driven and is aimed at the devel opment of methodologies to optimize the information derived from data. The increasing complexity of phenomena that scientists and engineers wish to model, together with our increased ability to gather, store and interrogate data, mean that the subjects of applied mathematics and statistics are increasingly required to work in conjunction in order to significantly progress understanding.
This article is concerned with a research program at the interface between these two disciplines, aimed at problems in differential equations where profusion of data and the sophisticated model combinetoproducethemathematicalproblem of obtaining informa tion from a probability measure on function space. In this context there is an array of problems with a common mathematical structure, namely that the probability measure in question is a change of measure from a Gaussian. We illustrate the wide-ranging ap plicability of this structure. For problems whose solution is determined by a probability measure on function space, information about the solution can be obtained by sampling from this probability measure. One way to do this is through the use of Markov chain Monte-Carlo(MCMC) methods. We showhowthe common mathematical structure of the aforementioned problems can be exploited in the design of effective MCMC methods.