Weak dependence and optimal quantitative self-normalized central limit theorems
Moritz Jirak
University of Vienna, Vienna, Austria
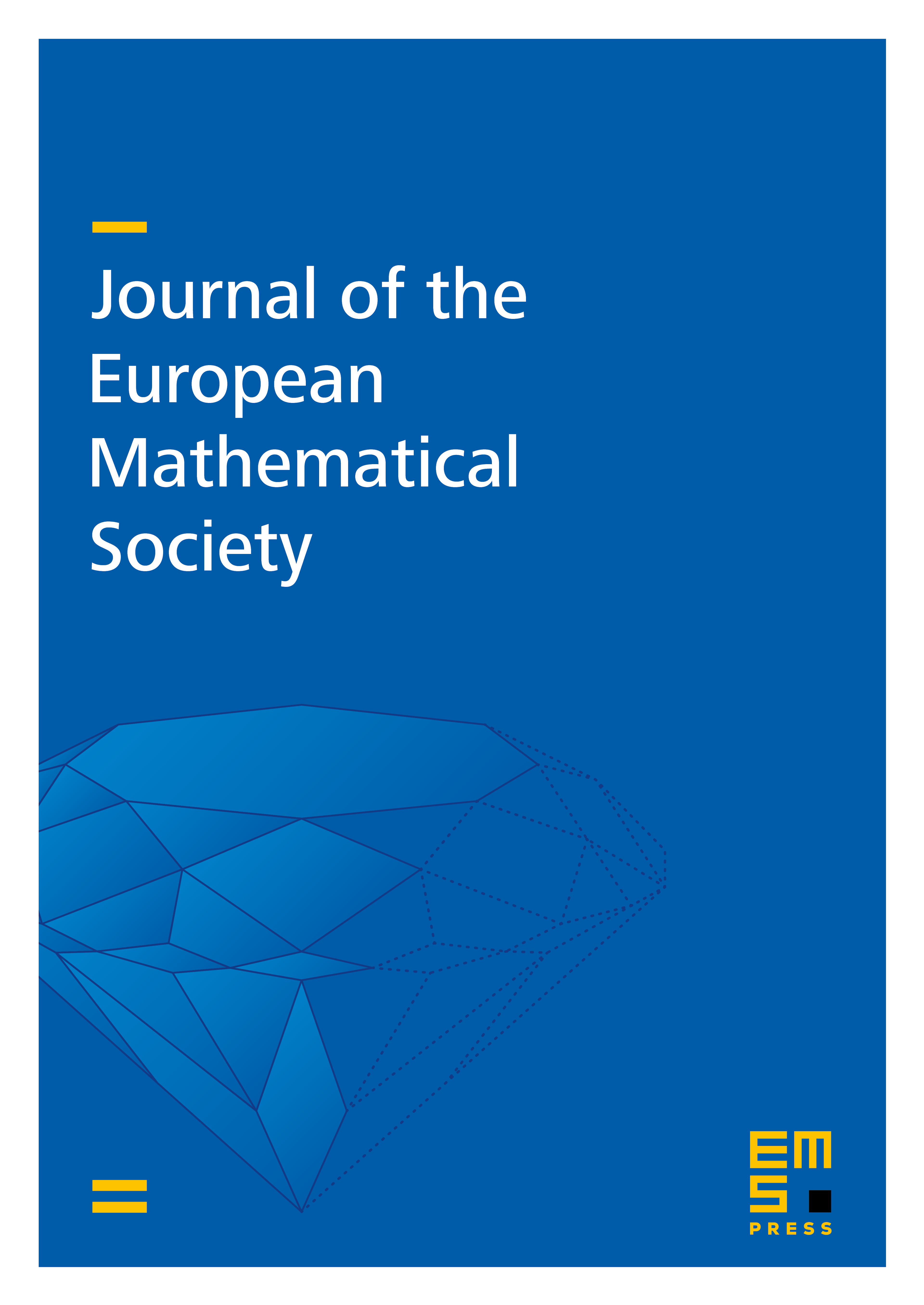
Abstract
Consider a stationary, weakly dependent sequence of random variables. Given only mild conditions, allowing for polynomial decay of the autocovariance function, we show a Berry–Esseen bound of optimal order for studentized (self-normalized) partial sums, both for the Kolmogorov and Wasserstein (and ) distances. The results show that in general, (minimax) optimal estimators of the long-run variance lead to suboptimal bounds in the central limit theorem, that is, the rate cannot be reached, refuting a popular belief in the literature. This can be salvaged by simple methods. We reveal that in order to maintain the optimal speed of convergence , simple over-smoothing within a certain range is necessary and sufficient. The setup contains many prominent dynamical systems and time series models, including random walks on the general linear group, products of positive random matrices, functionals of GARCH models of any order, functionals of dynamical systems arising from stochastic differential equations, iterated random functions and many more.
Cite this article
Moritz Jirak, Weak dependence and optimal quantitative self-normalized central limit theorems. J. Eur. Math. Soc. (2025), published online first
DOI 10.4171/JEMS/1573