Optimal and instance-dependent guarantees for Markovian linear stochastic approximation
Wenlong Mou
University of Toronto, Toronto, CanadaAshwin Pananjady
Georgia Institute of Technology, Atlanta, USAMartin J. Wainwright
Massachusetts Institute of Technology, Cambridge, USAPeter L. Bartlett
University of California, Berkeley, USA; Google DeepMind, Mountain View, USA
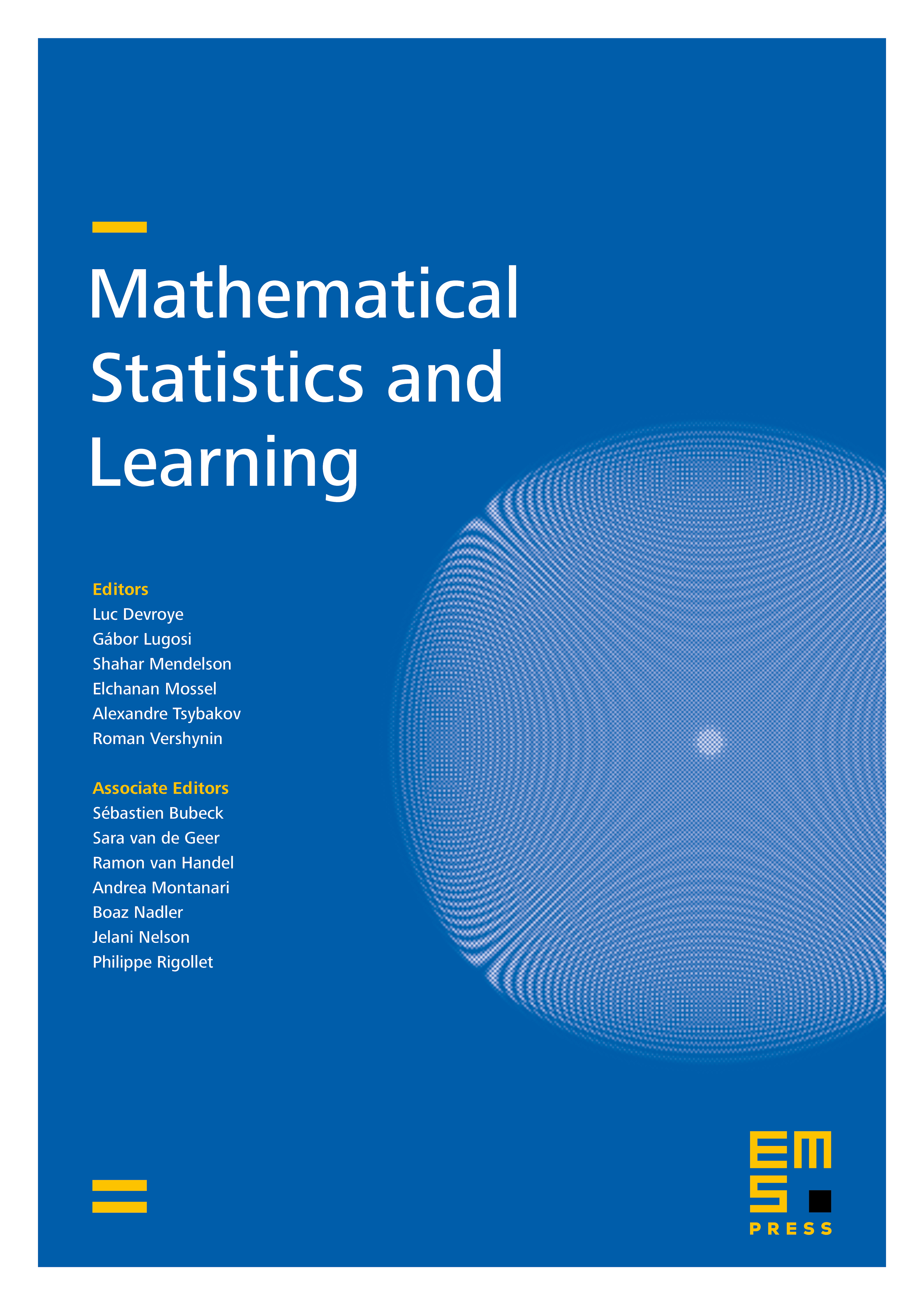
Abstract
We study stochastic approximation procedures for approximately solving a -dimensional linear fixed-point equation based on observing a trajectory of length from an ergodic Markov chain. We first exhibit a non-asymptotic bound of the order on the squared error of the last iterate of a standard scheme, where is a mixing time. We then prove a non-asymptotic instance-dependent bound on a suitably averaged sequence of iterates, with a leading term that matches the local asymptotic minimax limit, including sharp dependence on the parameters in the higher-order terms. We complement these upper bounds with a non-asymptotic minimax lower bound that establishes the instance-optimality of the averaged SA estimator. We derive corollaries of these results for policy evaluation with Markov noise—covering the family of algorithms for all —and linear autoregressive models. Our instance-dependent characterizations open the door to the design of fine-grained model selection procedures for hyperparameter tuning (e.g., choosing the value of when running the algorithm).
Cite this article
Wenlong Mou, Ashwin Pananjady, Martin J. Wainwright, Peter L. Bartlett, Optimal and instance-dependent guarantees for Markovian linear stochastic approximation. Math. Stat. Learn. 7 (2024), no. 1/2, pp. 41–153
DOI 10.4171/MSL/44