Partial recovery bounds for clustering with the relaxed -means
Christophe Giraud
Université Paris-Sud, Orsay, FranceNicolas Verzelen
Université de Montpellier, France
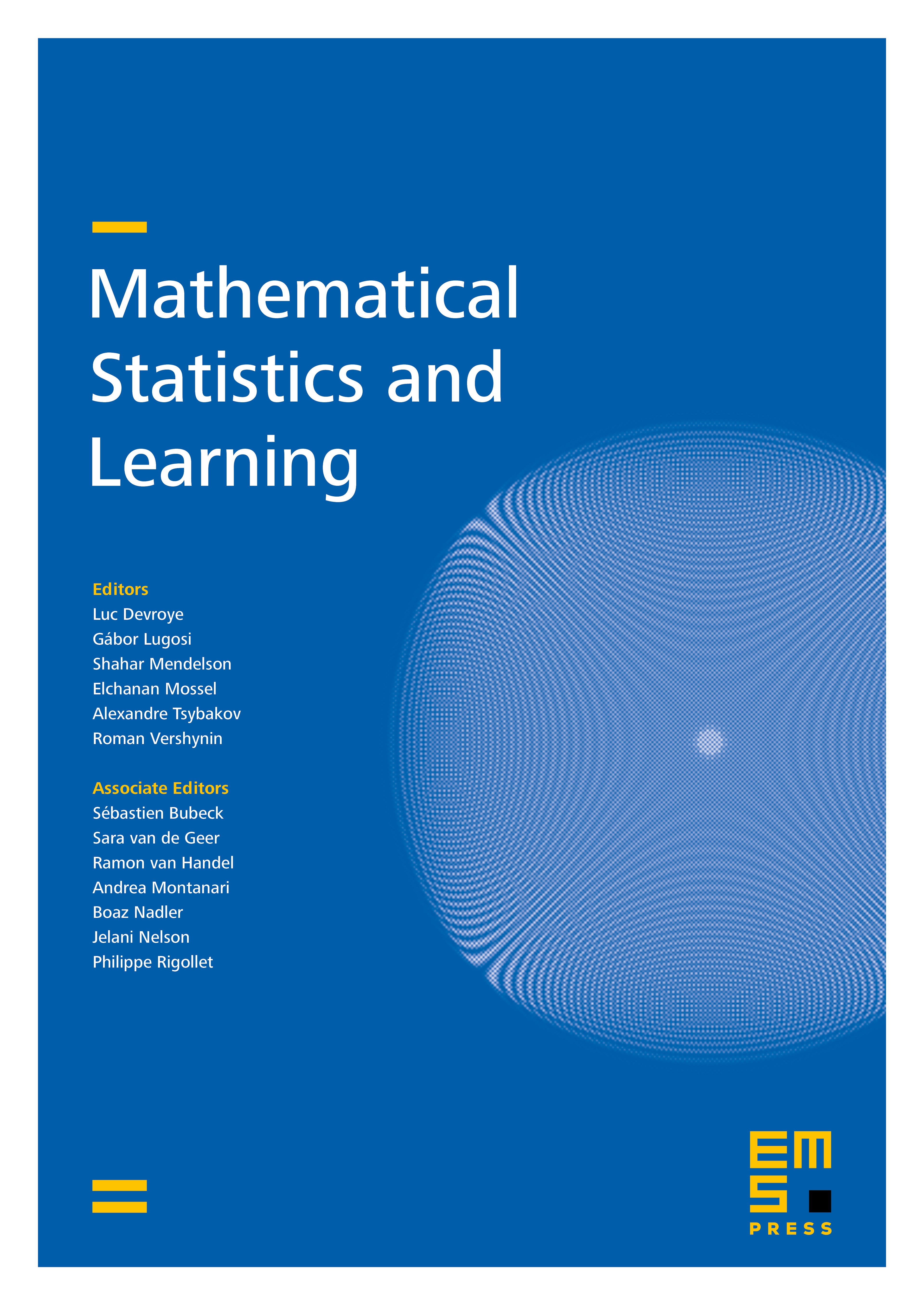
Abstract
We investigate the clustering performances of the relaxed -means in the setting of sub-Gaussian Mixture Model (sGMM) and Stochastic Block Model (SBM). After identifying the appropriate signal-to-noise ratio (SNR), we prove that the misclassification error decays exponentially fast with respect to this SNR. These partial recovery bounds for the relaxed -means improve upon results currently known in the sGMM setting. In the SBM setting, applying the relaxed -means SDP allows us to handle general connection probabilities whereas other SDPs investigated in the literature are restricted to the (dis-)assortative case (where within group probabilities are larger than between group probabilities). Again, this partial recovery bound complements the state-of-the-art results. All together, these results put forward the versatility of the relaxed -means.
Cite this article
Christophe Giraud, Nicolas Verzelen, Partial recovery bounds for clustering with the relaxed -means. Math. Stat. Learn. 1 (2018), no. 3/4, pp. 317–374
DOI 10.4171/MSL/8