Tensor denoising with trend filtering
Francesco Ortelli
ETH Zürich, SwitzerlandSara van de Geer
ETH Zürich, Switzerland
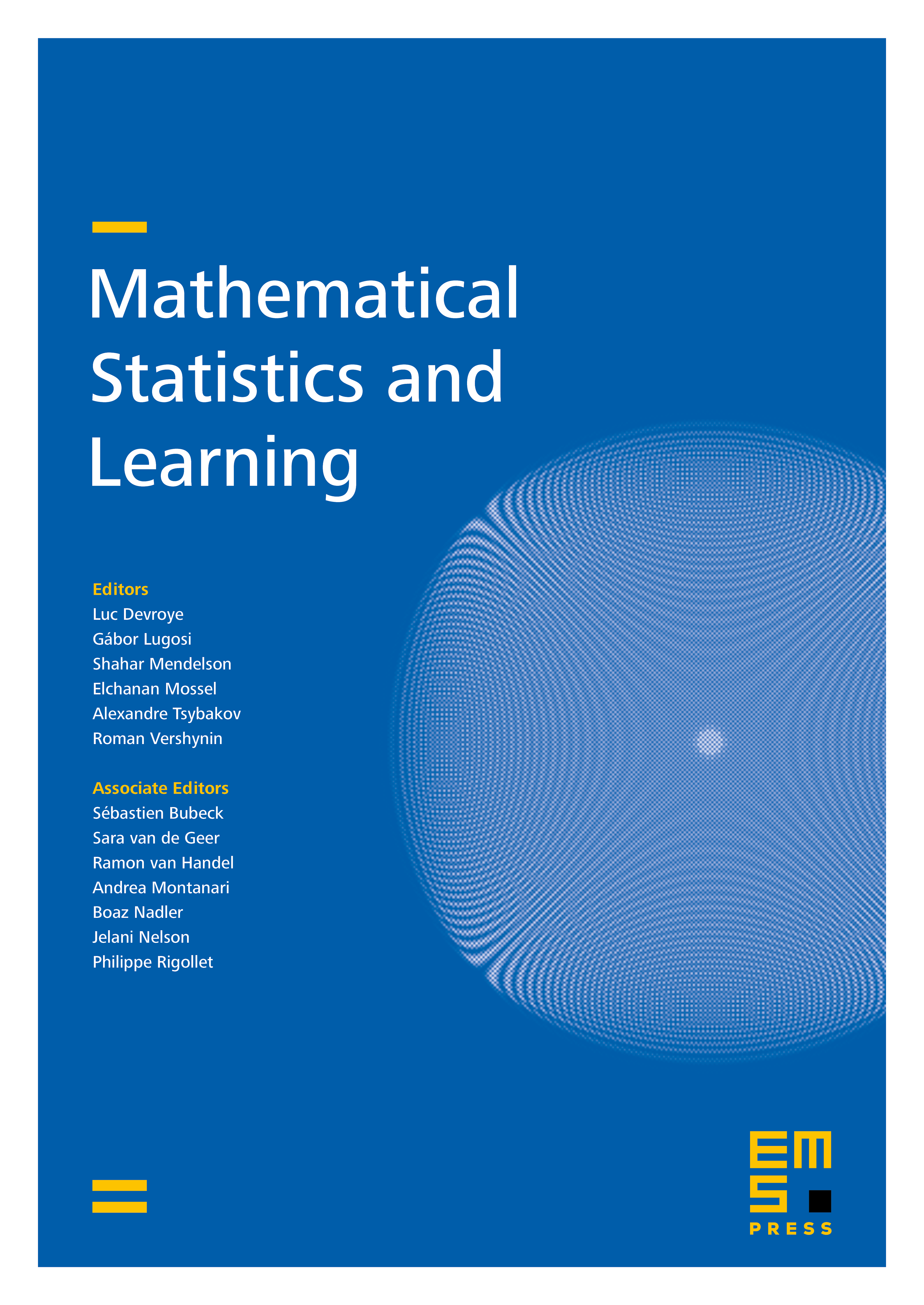
Abstract
We extend the notion of trend filtering to tensors by considering the -order Vitali variation – a discretized version of the integral of the absolute value of the -order total derivative. We prove adaptive -rates and not-so-slow -rates for tensor denoising with trend filtering.
For we prove that the -dimensional margin of a -dimensional tensor can be estimated at the -rate , up to logarithmic terms, if the underlying tensor is a product of -order polynomials on a constant number of hyperrectangles. For general we prove the -rate of estimation , up to logarithmic terms, where is the harmonic number.
Thanks to an ANOVA-type of decomposition we can apply these results to the lower dimensional margins of the tensor to prove bounds for denoising the whole tensor. Our tools are interpolating tensors to bound the effective sparsity for -rates, mesh grids for -rates and, in the background, the projection arguments by Dalalyan, Hebiri, and Lederer (2017).
Cite this article
Francesco Ortelli, Sara van de Geer, Tensor denoising with trend filtering. Math. Stat. Learn. 4 (2021), no. 1/2, pp. 87–142
DOI 10.4171/MSL/26