Distribution-free robust linear regression
Jaouad Mourtada
Institut Polytechnique de Paris, Palaiseau, FranceTomas Vaškevičius
University of Oxford, UKNikita Zhivotovskiy
ETH Zürich, Switzerland
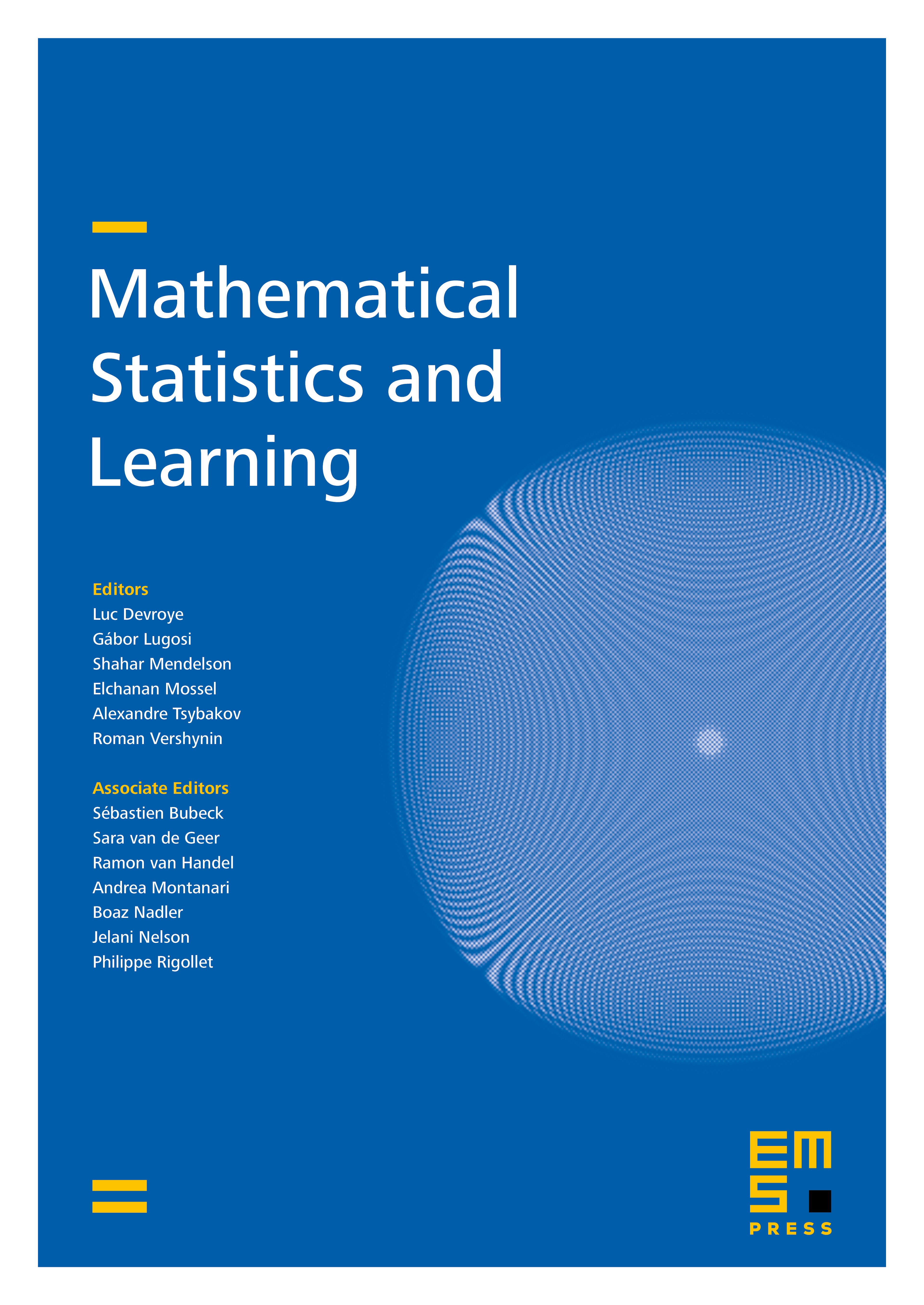
Abstract
We study random design linear regression with no assumptions on the distribution of the covariates and with a heavy-tailed response variable. In this distribution-free regression setting, we show that boundedness of the conditional second moment of the response given the covariates is a necessary and sufficient condition for achieving non-trivial guarantees. As a starting point, we prove an optimal version of the classical in-expectation bound for the truncated least squares estimator due to Györfi, Kohler, Krzyżak, and Walk. However, we show that this procedure fails with constant probability for some distributions despite its optimal in-expectation performance. Then, combining the ideas of truncated least squares, median-ofmeans procedures, and aggregation theory, we construct a non-linear estimator achieving excess risk of order with the optimal sub-exponential tail. While existing approaches to linear regression for heavy-tailed distributions focus on proper estimators that return linear functions, we highlight that the improperness of our procedure is necessary for attaining non-trivial guarantees in the distribution-free setting.
Cite this article
Jaouad Mourtada, Tomas Vaškevičius, Nikita Zhivotovskiy, Distribution-free robust linear regression. Math. Stat. Learn. 4 (2021), no. 3/4, pp. 253–292
DOI 10.4171/MSL/27