Statistische und Probabilistische Methoden der Modellwahl
James O. Berger
Duke University, Durham, United StatesHolger Dette
Ruhr-Universität Bochum, GermanyGábor Lugosi
Pompeu Fabra University, Barcelona, SpainAxel Munk
Georg-August-Universität Göttingen, Germany
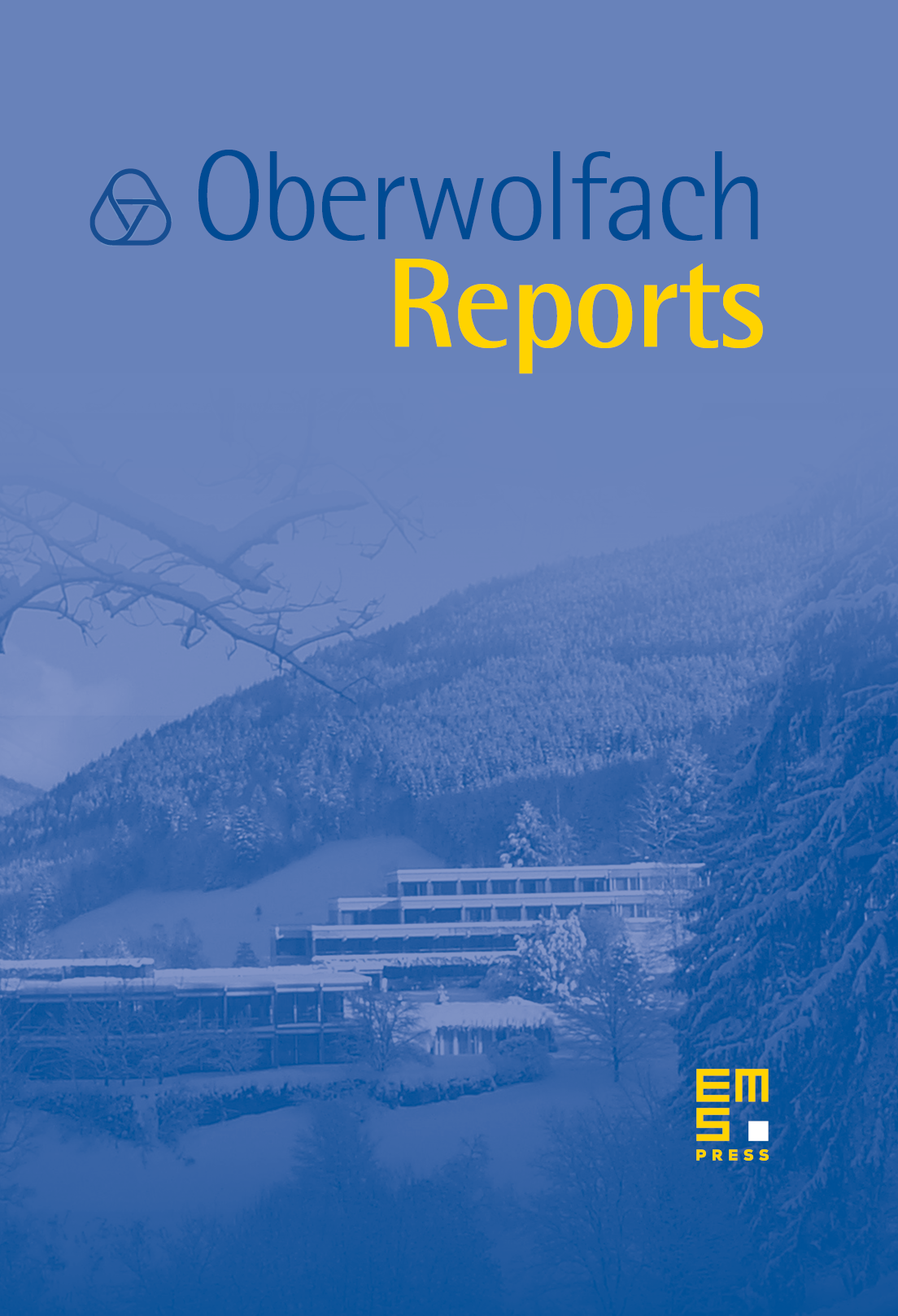
Abstract
In order to achieve our goal to enhance discussion between these communities, every day the conference was opened by a survey talk. Friday afternoon the conference has been closed by a discussion session.
1. Frequentist model selection and testing
Nils Hjort introduced in his talk the fundamental concept of a focused information criterion for model selction, which does not propagate a model per se, rather it reflects the more realistic situation, that specific aspects of a model should drive the model selction process. He adressed various questions related to this, e.g. robustness issues, or how do classical information criteria such as AIC or BIC behave from this perspectice. He gives strong evidenve by various examples that different models may result when focussing on different parameters of primary interest.
The issue of testing a model was adressed by various talks, N. Neumeyer used bootstrap techniques applied to residual processes whereas L. Györfi's criterion is based on the distance between densities. J. M. Loubes and N. Bissantz were concerned with model selection in inverse problems, i.e. for noisy integral operator equations. J.M. Loubes considers nonlinear operators which are locally linear and investigates convergence rates of penalized M-estimators. N. Bissantz focuses on distance based model testing and selection methods and discusses various applications in astrophysics. To this end, a general analyisis of numerical and statistical regularisation methods is given. Finally, he constructed uniform confidence bands in deconvolution problems which allow graphically to select a proper model. The problem of deconvolution was also highlighted by J. P Kreiss in the context of time series analysis. Conceptually related to N. Hjorts talk, J.K. Ghosh discussed different roles of different penalties in penalized likelihood model selection rules, making the case that the penalty used should depend on the goal (typically either prediction or selection of the best model) and that it is important to incorporate practical features such as growing model dimension in choosing penalties. L. Dümbgen was concerned with prediction regions in gaussian shift models. He suggested a solution but also pointed out that adaptive construction of prediction regions via a sequence of nested models is limited in various ways. This is in contrast to adaptive estimation. He discussed a 'no go' result on the asymptotic diameter of the confidence ball in the spirit of Li (1989).
Other talks included topics on Empirical process techniques for locally stationary processes by Rainer Dahlhaus and Universal principles, approximation and model choice by Patrick Laurie Davies and Local Parametric Methods in Nonparametric Regression by Vladimir Spokoiny.
2. Statistical learning theory and machine learning
Research on statistical learning theory and nonparametric classification has also been strongly represented by several attendants who partly or completely focus their research on these topics. Several talks have been given in these fields, offering a nice overview on some of the most active areas of investigation, such as oracle inequalities for penalized model selection, margin-based performance bounds, empirically calibrated penalties, model selection focusing on sparse solutions of corresponding optimization problems, convex aggregation of estimators, as well as some closely related issues emerging in density estimation, microarray analysis, etc.
Peter Bartlett (UC Berkeley) gave a survey talk on nonparametric classification based on empirical minimization of convex cost functionals, a subject that offers a theoretical framework for many successful classification algorithms, including boosting and support vector machines. Marten Wegkamp's talk (Florida State University) discussed a closely related problem of classification with a reject option. Another survey talk on a closely related subject was delivered by Sara van de Geer (ETH Zürich) who showed why empirical process theory and concentration inequalities play a crucial role in model selection problems for classification and nonparametric regression. Similarly to Prof. van de Geer, Vladimir Koltchinskii (Georgia Tech) also considered L1-type penalties that lead to sparse models and derived sharp oracle inequalities.
Both Alexandre Tsybakov (University of Paris 7) and Florentina Bunea (Florida State University) considered methods for convex aggregation of certain estimates for regression, and proved close-to-optimal performance bounds. László Györfi (Technical University of Budapest) presented a model selection method and a corresponding L1 performance bound for density estimation when the unknown density is assumed to be in one of an infinite sequence of “parametric” classes of densities.
Andrew Nobel (University of North Carolina) discussed algorithmic and probabilistic problems arising in some problems of data mining that can be modeled as searching for large homogeneous blocks in random matrices.
3. Bayesian model selection
In Bayesian model selection and BART, E. George and R. McCulloch gave a survey of the Bayesian approach to model selection, while giving an illustration (BART) that seems to have remarkable predictive properties in function estimation and variable selection. This was followed by Merlise Clyde, giving a talk on Bayesian nonparametric function estimation using overcomplete representations and Lévy random field priors. This focused on the novel notion in Bayesian analysis that simultaneous use of multiple bases for functions (leasing to overcompleteness) can be quite valuable in practice, because it can allow for extremely sparse representations of functions. The final Bayesian talk on Monday was by Christian Robert, on Prior choice and model selection. This highlighted the key issue faced by Bayesians in model choice, namely the choice of the prior distribution. Modern approaches to this issue were reviewed, and a new approach (based on a criterion of ‘matching’ between models) was introduced.
Later talks included A synthesis and unification of Bayes factors for model selection and hypothesis testing, by Luis Pericchi. This talk discussed the prominent role of training samples (or bootstrapping), in many modern model selection scenarios. Valen Johnson, in A note on the consistency and interpretation of Bayes factors based on test statistics considered the problem of developing easy to use Bayesian procedures as replacements for standard statistical procedures, such as chi-squared tests, t-tests, etc. He demonstrated how many Bayesian testing problems can be reduced to situations with only a one-dimensional unknown, which lend themselves to graphical description.
On the final day, the issue of multiple testing was addressed. This is one of the currently hottest areas of statistical and scientific research, and two talks were presented. M.-J. Bayarri gave a survey talk entitled Multiple testing: the problem and some solutions, which reviewed the connections between ‘false discovery rate,’ Bayesian posterior probabilities, and utility functions common in multiple testing scenarios. P. Müller followed with elaborations on the utility side, involving applications to significant problems in bioinformatics and clinical trials.
The final session in the workshop consisted of very short talks to give other participants (especially newer researchers) a chance to discuss their interests, and several Bayesian talks were presented. M. Bogdan presented Model selection approach to the problem of locating genes influencing quantitative traits, presenting a very nice generalization of BIC for a genetics problem. Katja Ickstadt presented Comparing classification procedures using misclassification rates, with an interesting application to determining genetic ‘snips.’ Angelika van der Linde spoke on Posterior predictive model choice, discussing a new asymptotic Bayesian approach to model choice, requiring a careful decomposition of entropy.
Closing Discussion Session: The workshop ended with a discussion session designed to identify key problems remaining to be addressed, and to identify key ways to bridge the gaps between the communities present at the workshop. The questions – together with short descriptions of the results of the discussion – are below.
- Do we all mean the same thing by the phrase model selection? Is it selection of a statistical model for the data, selection of a prediction function, or some averaged version of either?
- Conclusion: If prediction is the identified goal, then the various communities have the same view of model selection. Otherwise, interesting differences exist.
- Are fundamental problems of statistics and machine learning different? If they are the same, why are the commonly used techniques so different?
- Conclusion: Machine learning is concerned primarily with action and associated risk, and is less focused on inference, which is often viewed as the primarily goal of statistics.
- Discuss the parametric aspects of nonparametric models.
- Conclusion: Any nonparametric procedure is only good in certain finite dimensional regions of the nonparametric space.
- Is model selection fundamentally different when the true model is outside the class of models being considered?
- Conclusion: This is primarily an issue in Bayesian statistics, because the other viewpoints formulate the model class so that it is supposedly assured to contain the true model; there was, however dissension as to whether the latter was actually possible.
- How does information theory contribute to statistics?
- Conclusion: Notions such as ‘minimum description length’ are difficult to encode, and are arguably as difficult to implement as the more usual model/prior paradigm.
- Given that regularization is very related to Bayesian analysis,
- Do oracle or risk inequalities tell us about performance of Bayesian procedures? In practice? For (growing) finite sample size? Asymptotically?
- Can regularization results help Bayesians in choosing priors? Do oracle based convergence rates relate to optimal objective priors?
- How do oracle inequalities relate to AIC, BIC, ?
- Conclusion: AIC and BIC are not derivable as oracle inequalities. Indeed, only if the constants in oracle inequalities are essentially one (i.e., the inequalities are exact in some regions), can there be a hope that oracle inequalities and Bayesian analysis will coincide. The other questions are fundamentally unknown issues for future study.
Cite this article
James O. Berger, Holger Dette, Gábor Lugosi, Axel Munk, Statistische und Probabilistische Methoden der Modellwahl. Oberwolfach Rep. 2 (2005), no. 4, pp. 2611–2704
DOI 10.4171/OWR/2005/47