Statistical Inference for Complex Time Series Data
Rainer Dahlhaus
Universität Heidelberg, GermanyOliver Linton
University of Cambridge, United KingdomWei-Biao Wu
University of Chicago, USAQiwei Yao
London School of Economics, UK
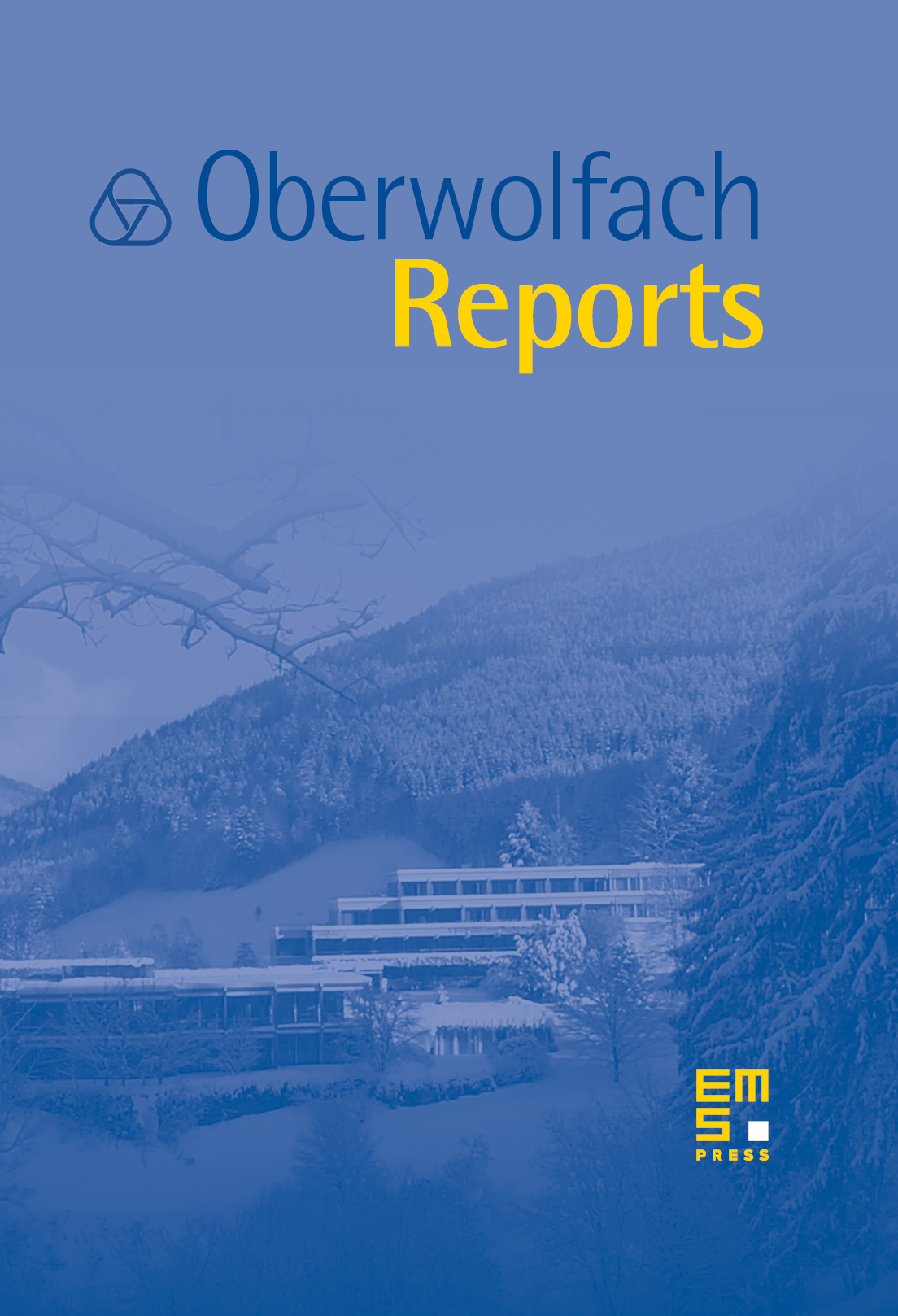
Abstract
During recent years the focus of scientific interest has turned from low dimensional stationary time series to nonstationary time series and high dimensional time series. In addition new methodological challenges are coming from high frequency finance where data are recorded and analyzed on a millisecond basis. The three topics “nonstationarity”, “high dimensionality” and “high frequency” are on the forefront of present research in time series analysis. The topics also have some overlap in that there already exists work on the intersection of these three topics, e.g. on locally stationary diffusion models, on high dimensional covariance matrices for high frequency data, or on multivariate dynamic factor models for nonstationary processes. The aim of the workshop was to bring together researchers from time series analysis, nonparametric statistics, econometrics and empirical finance to work on these topics. This aim was successfully achieved and the workshops was very well attended.
Cite this article
Rainer Dahlhaus, Oliver Linton, Wei-Biao Wu, Qiwei Yao, Statistical Inference for Complex Time Series Data. Oberwolfach Rep. 10 (2013), no. 3, pp. 2749–2823
DOI 10.4171/OWR/2013/48