Statistical and Computational Aspects of Learning with Complex Structure
Sara van de Geer
ETH Zentrum, Zürich, SwitzerlandMarkus Reiß
Humboldt-Universität Berlin, GermanyPhilippe Rigollet
Massachusetts Institute of Technology, Cambridge, USA
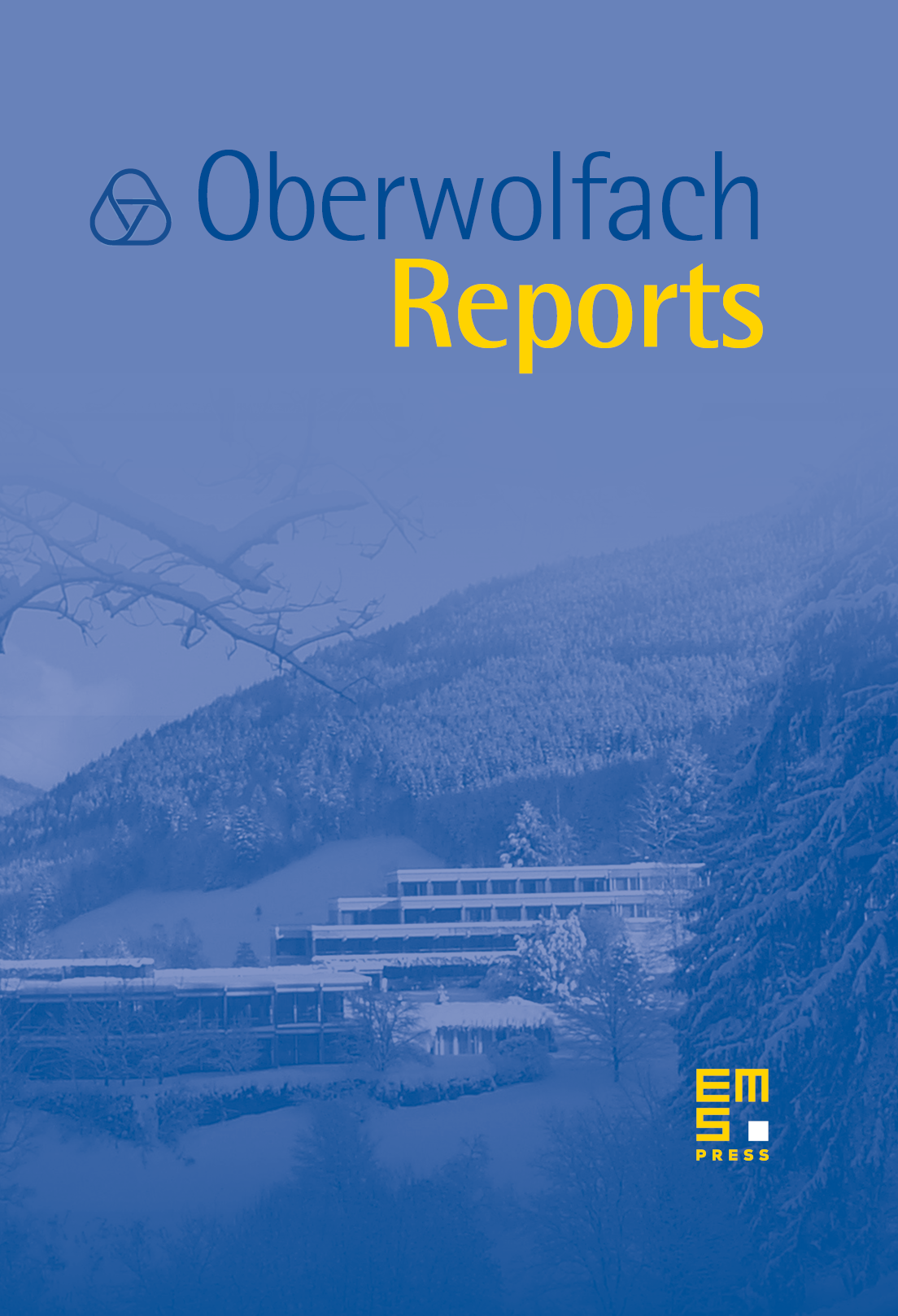
Abstract
The recent explosion of data that is routinely collected has led scientists to contemplate more and more sophisticated structural assumptions. Understanding how to harness and exploit such structure is key to improving the prediction accuracy of various statistical procedures. The ultimate goal of this line of research is to develop a set of tools that leverage underlying complex structures to pool information across observations and ultimately improve statistical accuracy as well as computational efficiency of the deployed methods. The workshop focused on recent developments in regression and matrix estimation under various complex constraints such as physical, computational, privacy, sparsity or robustness. Optimal-transport based techniques for geometric data analysis were also a main topic of the workshop.
Cite this article
Sara van de Geer, Markus Reiß, Philippe Rigollet, Statistical and Computational Aspects of Learning with Complex Structure. Oberwolfach Rep. 16 (2019), no. 2, pp. 1309–1356
DOI 10.4171/OWR/2019/22