Reassessing the Paradigms of Statistical Model-Building
Ursula Gather
Universität Dortmund, GermanyPeter G. Hall
University of Melbourne, AustraliaHans Rudolf Künsch
University of Zürich, Switzerland
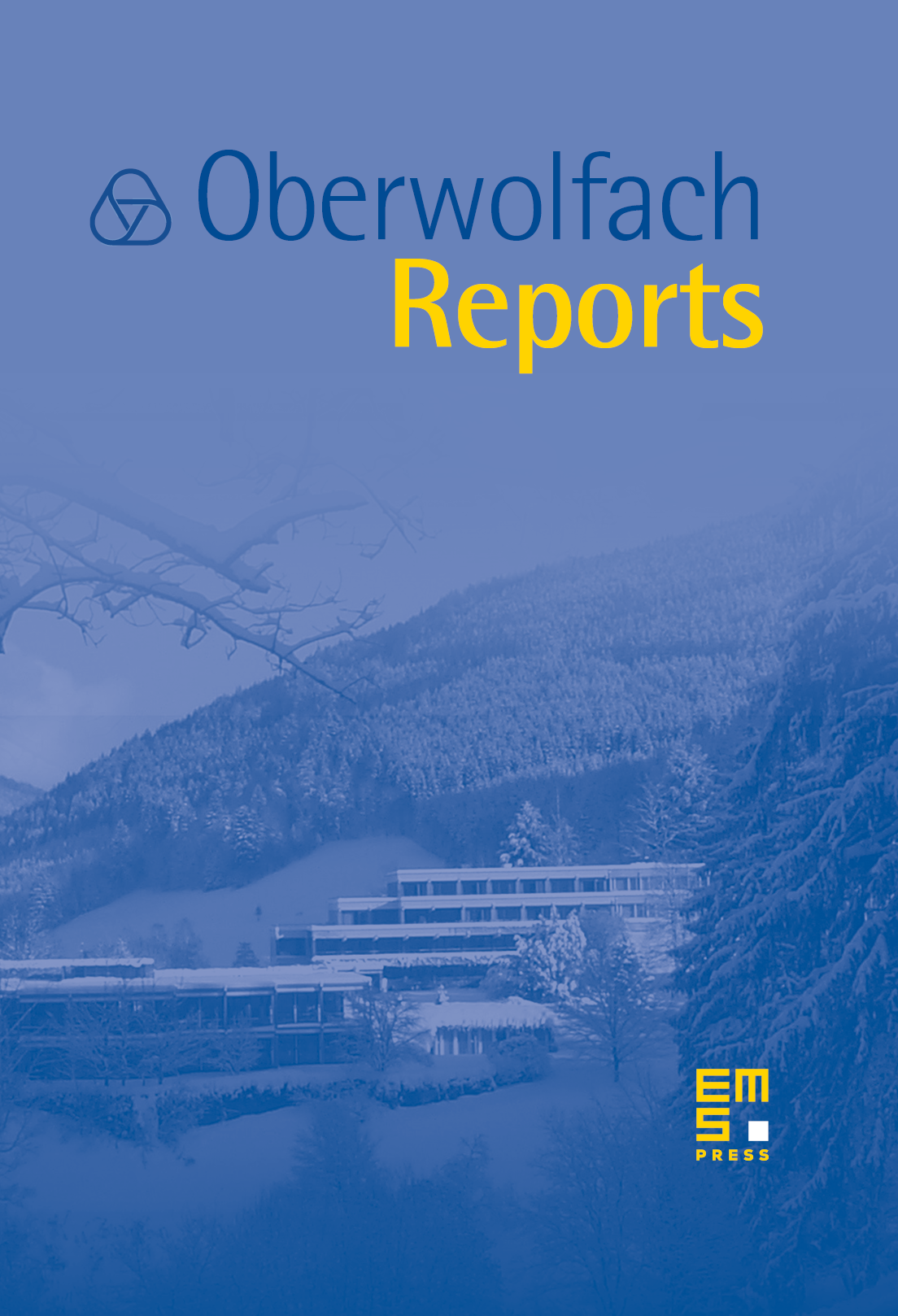
Abstract
The development of statistics during the last century has involved largely disjoint paradigms. Sometimes these have been complementary, for example in the case of Bayesian and frequentist methodologies. In other instances they have been overlapping, e.g. model-selection methods such as minimum description length methods and Akaike's information criterion; or evolutionary, e.g. parametric, semiparametric and nonparametric approaches; or developed along similar lines, e.g. parametric and nonparametric approaches to likelihood; or related in other ways, e.g. dimension-reduction methods and techniques for analysing high-dimensional data. The mathematical theory behind these techniques is especially complex and difficult. One example is the understanding and harnessing of the geometry of likelihood, which is still a major task for theoretical statisticians.
Finding a statistical model may include graphical representation of the data, calculation of relevant statistics, checking of putative models against the data, and assessment of possible outliers or serial correlations. In other cases, such as in nonparametric regression, the family of models is so large that a major aspect of the problem is choosing a particular model from a very large class, for example in the context of sparsity.
It is often only at the final stage of model specification that such formalised strategies are employed. Examples include Akaike's information criterion (AIC), Bayes information criterion (BIC), minimum description length and stochastic complexity (MDL) and cross-validation.
When statistical model selection is framed in a mathematical setting, it often arises as an optimisation problem, and has many points of contact with applied mathematics. The constraints, and hence the objective function, are determined by the paradigm. In this context the type of topology (strong or weak), the methods for computation and other mathematical issues play major roles. Statisticians are forced to apply and also to develop mathematical theory in order to find the techniques and concepts they need to understand the complex, real-world problems that motivate advances in their subject.
In the past the paradigms of statistical model building were developed separately. In the future, multiple paradigms will have to be used simultaneously. Indeed, many frontier problems in statistics today already involve several different concepts simultaneously. For example, techniques for analysing complex, high-dimensional data sets often use methods for complexity measurement, dimension reduction and classification. The demand for multiple paradigms in statistical model building was a major motivator of the workshop.
Thus, the workshop drew together statisticians working on the development and application of statistical model building, with the aim of critiquing different approaches, assessing their usefulness, developing new techniques, and mapping future directions for research.
The workshop was well attended, with 45 participants from all over the world, among them many young researchers. We were able to bring together experts from different fields of statistics: Bayesian methods, machine learning, likelihood theory, minimum description length and others.
Each morning, especially towards the beginning of the workshop, somewhat longer lectures were presented by senior researchers. The opening lecture was given by L. D. Brown, followed by other review-type presentations during the first three days for example by A.P. Dawid, S. Fienberg, R. Beran, R. Shibata, J. Rissanen, P. L. Davies and A.B. Tsybakov.
These lectures gave rise to a lively floor discussion on Wednesday evening, on the very meaning of statistical paradigms and on the new tasks for statistics in finding methods extracting important information from data. Particular attention was focused on challenging statistical problems emerging from new research areas, arising for example in the life sciences, physics, the social sciences, etc.
To characterise better these new challenges, which are often associated with new data structures, talks of more applied type were presented during the last two days, for example by G. Winkler, R. Carroll, A. Welsh, J. Ramsay and P. Hall. These yielded further discussion, and also new research cooperations among the participants.
Cite this article
Ursula Gather, Peter G. Hall, Hans Rudolf Künsch, Reassessing the Paradigms of Statistical Model-Building. Oberwolfach Rep. 4 (2007), no. 4, pp. 2943–3012
DOI 10.4171/OWR/2007/50