Statistics meets Machine Learning
Fadoua Balabdaoui
ETH Zürich, SwitzerlandLutz Dümbgen
Universität Bern, SwitzerlandKlaus-Robert Müller
Fraunhofer Institut, Berlin, GermanyRichard Samworth
University of Cambridge, UK
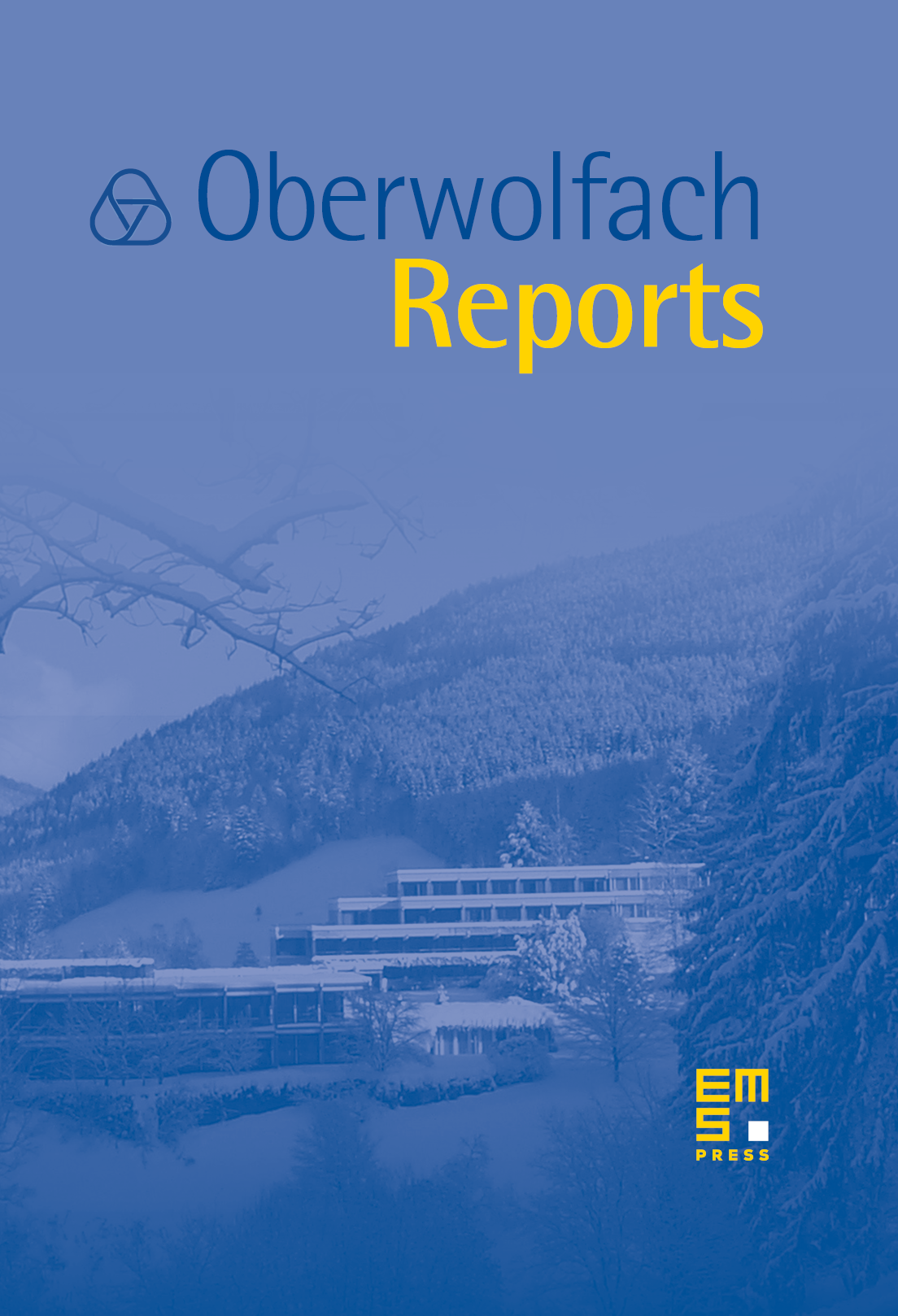
Abstract
Theory and application go hand in hand in most areas of statistics. In a world flooded with huge amounts of data waiting to be analyzed, classified and transformed into useful outputs, the designing of fast, robust and stable algorithms has never been as important as it is today. On the other hand, irrespective of whether the focus is put on estimation, prediction, classification or other purposes, it is equally crucial to provide clear guarantees that such algorithms have strong theoretical guarantees. Many statisticians, independently of their original research interests, have become increasingly aware of the importance of the numerical needs faced in numerous applications including gene expression profiling, health care, pattern and speech recognition, data security, marketing personalization, natural language processing, to name just a few.
The goal of this workshop is twofold: (a) exchange knowledge on successful algorithmic approaches and discuss some of the existing challenges, and (b) to bring together researchers in statistics and machine learning with the aim of sharing expertise and exploiting possible differences in points of views to obtain a better understanding of some of the common important problems.
Cite this article
Fadoua Balabdaoui, Lutz Dümbgen, Klaus-Robert Müller, Richard Samworth, Statistics meets Machine Learning. Oberwolfach Rep. 17 (2020), no. 1, pp. 231–272
DOI 10.4171/OWR/2020/4