Mini-Workshop: Time Series with Sudden Structural Changes
Richard A. Davis
Columbia University, New York, USAJürgen Franke
Universität Kaiserslautern, Germany
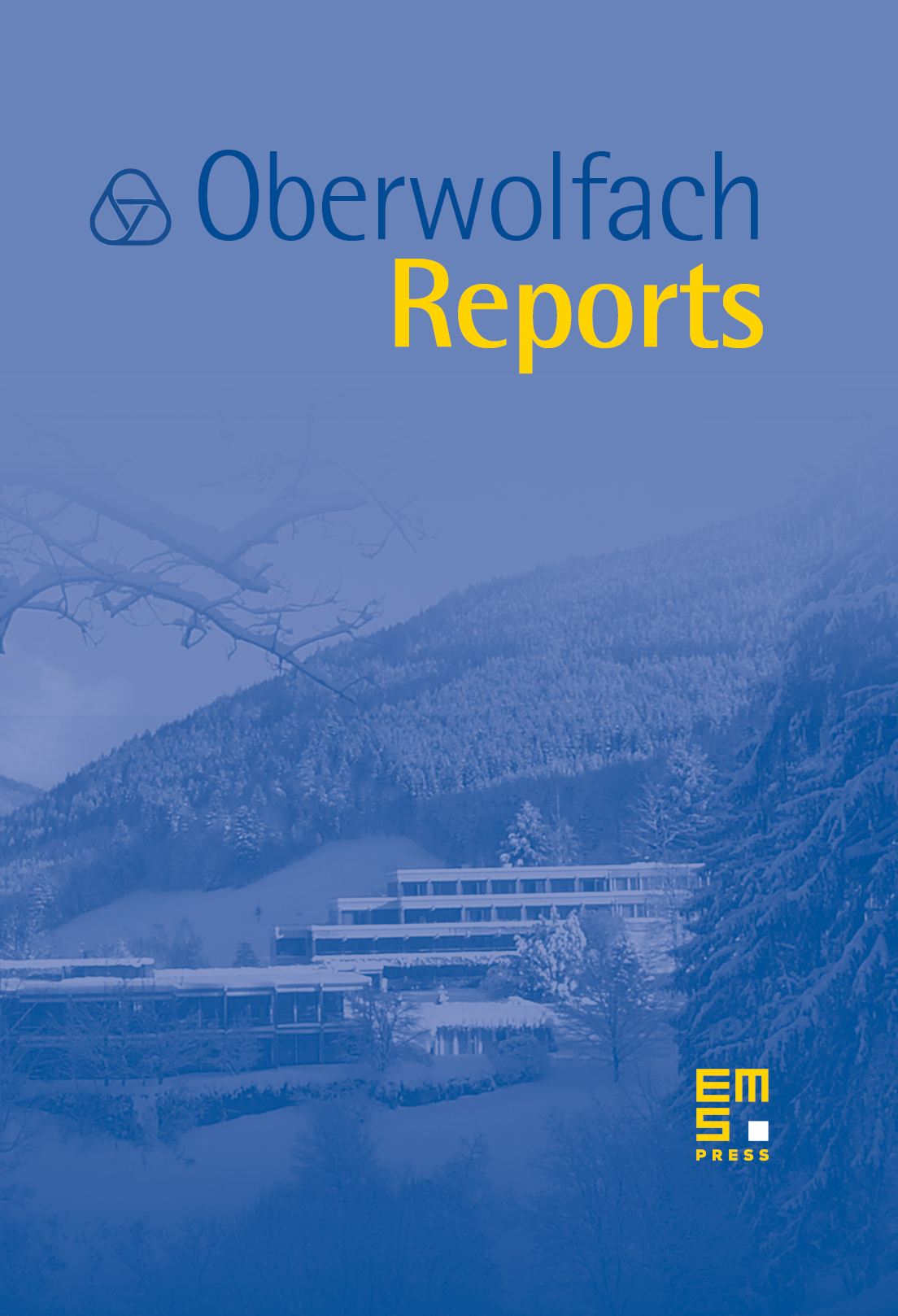
Abstract
Time series with nonstationarities can be found in a variety of applications from economics to engineering to biological and medical sciences to geosciences. During the past 20 years, much effort in investigating nonstationary time series has been focused on models with slowly changing structure. Such examples include autoregressive processes with slowly changing parameters and, more generally, locally stationary processes with a time-dependent spectrum. Research on these models has led to considerable insight into the structure of stochastic processes as well as parametric and nonparametric statistical methods. In particular, the fact that time series data are no longer assumed to be identically distributed has resulted in some challenging and interesting statistical problems.
Typically locally stationary models are not well suited to cover the situation in which a time series may experience a sudden change in its dynamics. That is, there may be changes in the mean, variance, dependence structure, or some other feature that occur at random times. Often it is reasonable to assume that the time series is stationary between these changepoints. This assumption is tacitly made in the applications of classical changepoint analysis techniques to stochastic processes of dependent data which have been proposed recently. It also forms the basis for segmentation techniques which partition the time axis such that the data in each subinterval may be modeled by a stationary process. Another approach explicitly takes the piecewise stationarity into account in the modeling step. There, the sudden changes in the dynamic structure of the data-generating process are controlled by a hidden Markov chain with finitely many states resulting in hidden Markov models, or, more generally, in Markov switching models. Such models are becoming increasingly popular in the analysis of economic, environmental and other data.
Some progress has been recently made in the theory regarding the stochastic structure of such piecewise stationary time series models as well as regarding the properties of parametric and nonparametric statistical procedures for estimating the system characteristics, of estimating locations of changepoints and of reconstructing the unobservable state process, i.e. of finding an appropriate segmentation of the data. Much of this work also requires the development of algorithms that are numerical feasible for carrying out these procedures. Nevertheless, there are still many open questions, and one of the main goals of the workshop beyond the exchange of current results between different statistical communitites working on those problems, was to join forces to discuss ideas on how to attack various open problems and to investigate the relations between different approaches.
A combination of review talks as well as presentations of recent progress in theory and of challenging applications formed the basis for the exchange of ideas. Altogether, there were 16 talks which invariably were accompanied by a very lively discussion. The presented results and the raised questions served as inspiration for future research and as stimulation for joint projects. An open problem session also contributed to the fruitful communication between the different research communities. At the end of the conference, the participants decided to continue the efforts started at the workshop and to further communication by creating a website which serves as a platform for researchers working on time series with change points.
Cite this article
Richard A. Davis, Jürgen Franke, Mini-Workshop: Time Series with Sudden Structural Changes. Oberwolfach Rep. 5 (2008), no. 1, pp. 557–586
DOI 10.4171/OWR/2008/12