Computational Inverse Problems for Partial Differential Equations
Liliana Borcea
University of Michigan, Ann Arbor, USAThorsten Hohage
Universität Göttingen, GermanyBarbara Kaltenbacher
Alpen-Adria-Universität, Klagenfurt, Austria
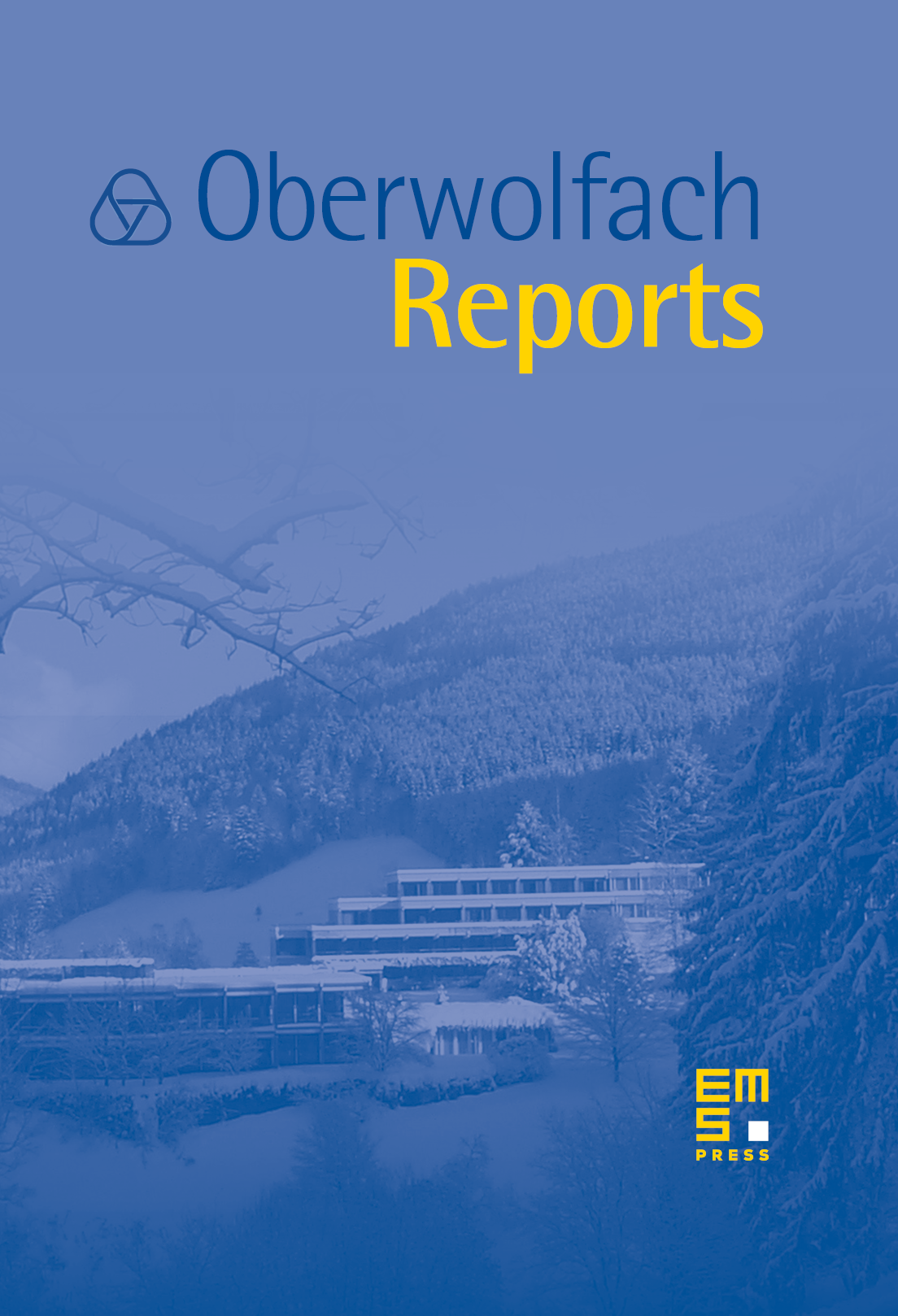
Abstract
Inverse problems in partial differential equations (PDEs) consist in reconstructing some part of a PDE such as a coefficient, a boundary condition, an initial condition, the shape of a domain, or a singularity from partial knowledge of solutions to the PDE. This has numerous applications in nondestructive testing, medical imaging, seismology, and optical imaging. Whereas classically mostly boundary or far field data of solutions to deterministic PDEs were considered, more recently also statistical properties of solutions to random PDEs have been studied. The study of numerical reconstruction methods of inverse problems in PDEs is at the interface of numerical analysis, PDE theory, functional analysis, statistics, optimization, and differential geometry. This workshop has mainly addressed five related topics of current interest: model reduction, control-based techniques in inverse problems, imaging with correlation data of waves, fractional diffusion, and model-based approaches using machine learning.
Cite this article
Liliana Borcea, Thorsten Hohage, Barbara Kaltenbacher, Computational Inverse Problems for Partial Differential Equations. Oberwolfach Rep. 17 (2020), no. 4, pp. 1903–1954
DOI 10.4171/OWR/2020/39