Variational regularization in inverse problems and machine learning
Martin Burger
Friedrich-Alexander-Universität Erlangen-Nürnberg, Germany
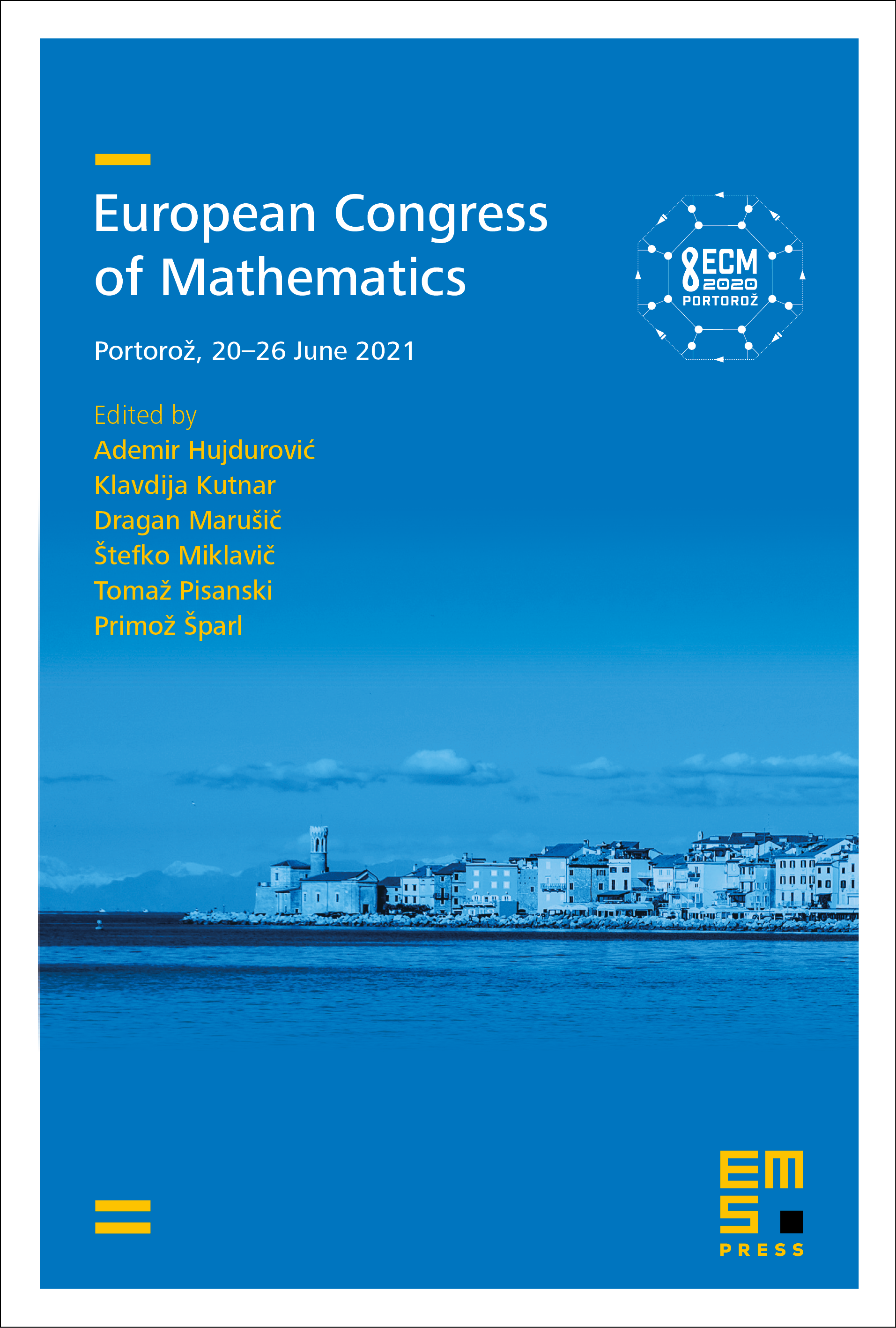
This book chapter is published open access.
Abstract
This paper discusses basic results and recent developments on variational regularization methods, as developed for inverse problems. In a typical setup we review basic properties needed to obtain a convergent regularization scheme and further discuss the derivation of quantitative estimates respectively the needed ingredients such as Bregman distances for convex functionals.
In addition to the approach developed for inverse problems, we will also discuss variational regularization in machine learning and work out some connections to the classical regularization theory. In particular we will discuss a reinterpretation of machine learning problems in the framework of regularization theory and a reinterpretation of variational methods for inverse problems in the framework of risk minimization. Moreover, we establish some previously unknown connections between error estimates in Bregman distances and generalization errors.