Gaussianity and typicality in matrix distributional semantics
Sanjaye Ramgoolam
Queen Mary University of London, UK; University of the Witwatersrand, Johannesburg, South AfricaMehrnoosh Sadrzadeh
University College London, UKLewis Sword
Queen Mary University of London, UK
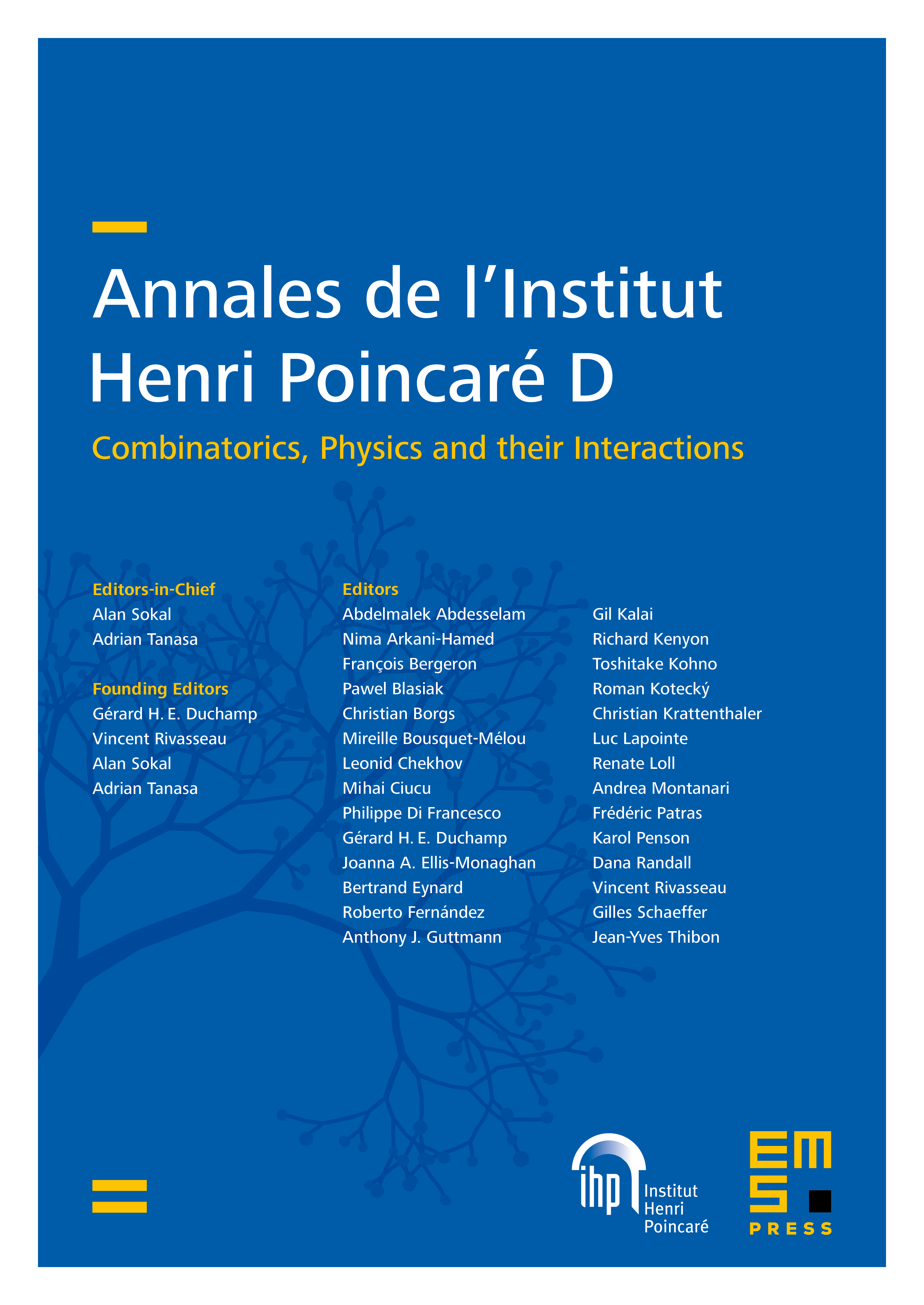
Abstract
Constructions in type-driven compositional distributional semantics associate large collections of matrices of size to linguistic corpora. We develop the proposal of analysing the statistical characteristics of this data in the framework of permutation invariant matrix models. The observables in this framework are permutation invariant polynomial functions of the matrix entries, which correspond to directed graphs. Using the general 13-parameter permutation invariant Gaussian matrix models recently solved, we find, using a dataset of matrices constructed via standard techniques in distributional semantics, that the expectation values of a large class of cubic and quartic observables show high Gaussianity at levels between 90 to 99 percent. Beyond expectation values, which are averages over words, the dataset allows the computation of standard deviations for each observable, which can be viewed as a measure of typicality for each observable. There is a wide range of magnitudes in the measures of typicality. The permutation invariant matrix models, considered as functions of random couplings, give a very good prediction of the magnitude of the typicality for different observables. We find evidence that observables with similar matrix model characteristics of Gaussianity and typicality also have high degrees of correlation between the ranked lists of words associated to these observables.
Cite this article
Sanjaye Ramgoolam, Mehrnoosh Sadrzadeh, Lewis Sword, Gaussianity and typicality in matrix distributional semantics. Ann. Inst. Henri Poincaré Comb. Phys. Interact. 9 (2022), no. 1, pp. 1–45
DOI 10.4171/AIHPD/112