On polynomial-time computation of high-dimensional posterior measures by Langevin-type algorithms
Richard Nickl
University of Cambridge, UKSven Wang
Massachusetts Institute of Technology, Cambridge, USA
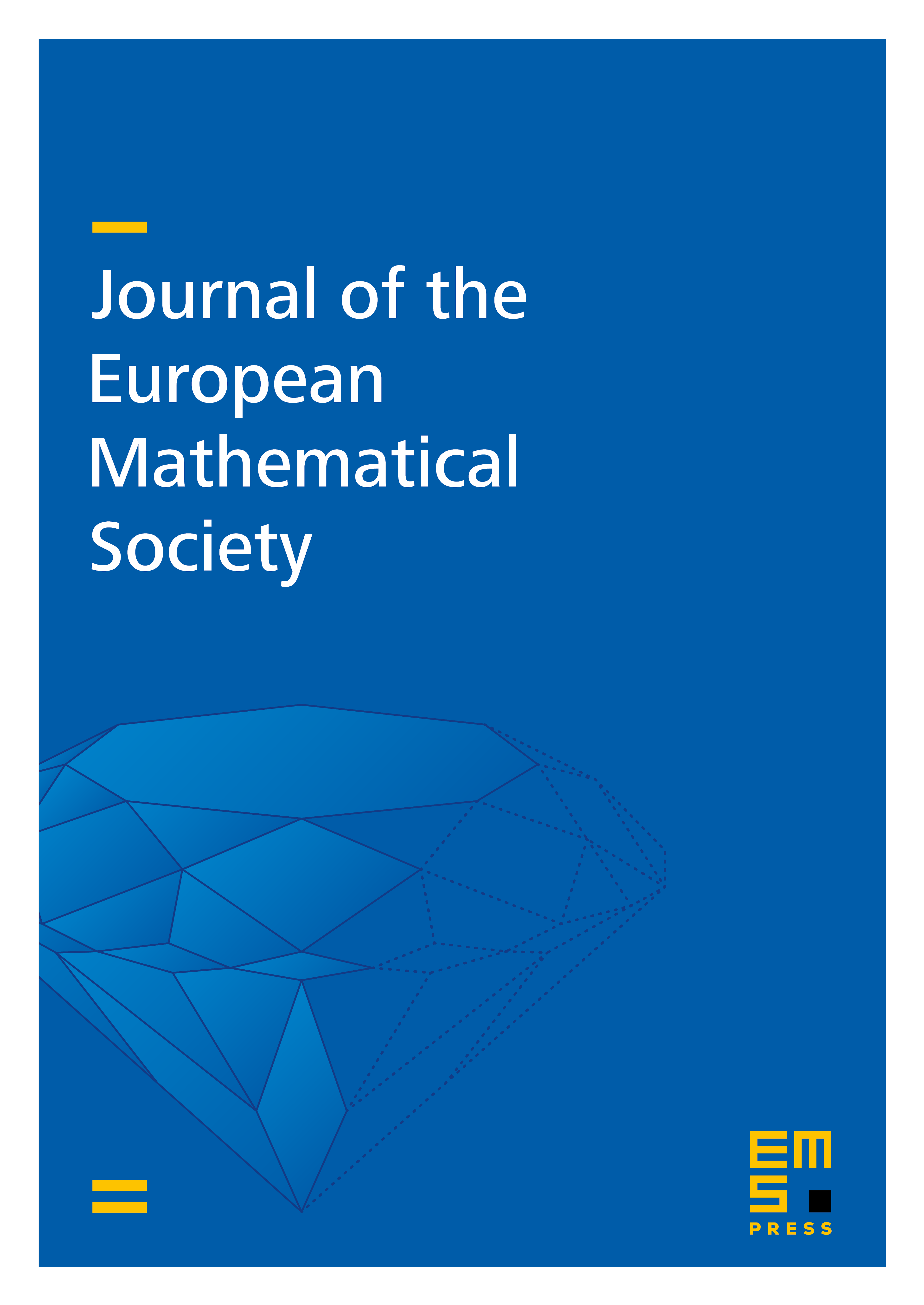
Abstract
The problem of generating random samples of high-dimensional posterior distributions is considered. The main results consist of non-asymptotic computational guarantees for Langevin-type MCMC algorithms which scale polynomially in key quantities such as the dimension of the model, the desired precision level, and the number of available statistical measurements. As a direct consequence, it is shown that posterior mean vectors as well as optimisation based maximum a posteriori (MAP) estimates are computable in polynomial time, with high probability under the distribution of the data. These results are complemented by statistical guarantees for recovery of the ground truth parameter generating the data.
Our results are derived in a general high-dimensional non-linear regression setting (with Gaussian process priors) where posterior measures are not necessarily log-concave, employing a set of local ‘geometric’ assumptions on the parameter space, and assuming that a good initialiser of the algorithm is available. The theory is applied to a representative non-linear example from PDEs involving a steady-state Schrödinger equation.
Cite this article
Richard Nickl, Sven Wang, On polynomial-time computation of high-dimensional posterior measures by Langevin-type algorithms. J. Eur. Math. Soc. 26 (2024), no. 3, pp. 1031–1112
DOI 10.4171/JEMS/1304