Notes on computational-to-statistical gaps: predictions using statistical physics
Afonso Bandeira
Courant Institute, New York University, USAAmelia Perry
Massachusetts Institute of Technology, Cambridge, USAAlexander S. Wein
Courant Institute, New York University, USA
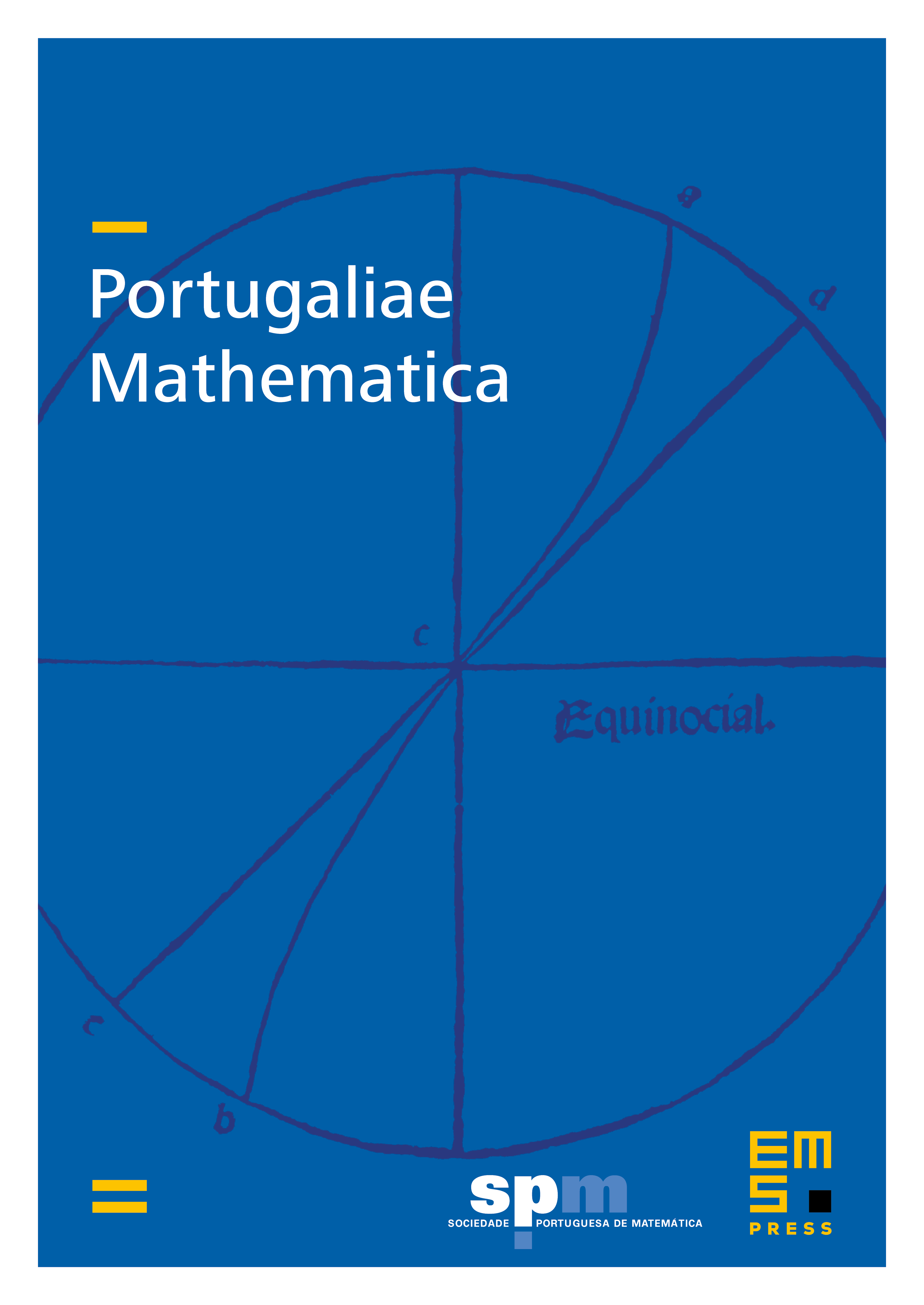
Abstract
In these notes we describe heuristics to predict computational-to-statistical gaps in certain statistical problems. These are regimes in which the underlying statistical problem is information-theoretically possible although no efficient algorithm exists, rendering the problem essentially unsolvable for large instances. The methods we describe here are based on mature, albeit non-rigorous, tools from statistical physics.
Cite this article
Afonso Bandeira, Amelia Perry, Alexander S. Wein, Notes on computational-to-statistical gaps: predictions using statistical physics. Port. Math. 75 (2018), no. 2, pp. 159–186
DOI 10.4171/PM/2014